Array Labs: 3D Mapping Earth from Space
Satellite Clusters and the Quest for the Holy Grail of EO
Welcome to the 78 newly Not Boring people who have joined us since last Tuesday! If you haven’t subscribed, join 211,943smart, curious folks by subscribing here:
Today’s Not Boring, the whole thing, is brought to you by… Array Labs
If you get as fired up reading this essay as I did writing it, you can simply go build radar satellite clusters to map the Earth in 3D by joining Array Labs:
Hi friends 👋,
Happy Tuesday! It’s fall in New York City, the Eagles looked great on Monday Night Football, and I get to bring you a deep dive that’s been melting my brain for weeks.
If I’ve spoken with you over the past few weeks, chances are I’ve told you about Array Labs. It was designed for me. A deeply Hard Startup, riding exponential technology curves, to build distributed systems for space, to enable businesses on Earth, with a strategy as thoughtful as its hardware.
Nothing makes me happier than banging my head against a complex company like Array for weeks to understand it well enough to share it with all of you. This is a longer essay that I’ve written in a while, because fully appreciating what Array is trying to do requires some knowledge of the earth observation market, how satellites work, how radars work, business strategy, the self-driving car market, and more.
This is a Sponsored Deep Dive. I’ve always said that I’d only write them on companies I’d want to invest in — and I hope to invest in Array Labs when they raise next — and as Not Boring Capital’s thesis has tightened to Hard Startups that can bend the world’s trajectory upwards, so has the deep dive bar. Array clears that bar by hundreds of kilometers. You can read more about how I choose which companies to do deep dives on, and how I write them here.
So throw on some appropriate music…
And let’s get to it.
Array Labs: 3D Mapping Earth from Space
A real-time, 3D map of the world is the holy grail of earth observation (EO).
Possessing such a map would enable new applications and technologies, from self-driving cars to augmented reality. It would improve climate monitoring, disaster response, construction management, resource management, and even urban planning.
Access to a real-time global 3D map would confer significant strategic and economic advantages to whoever possesses it. The US government has spent untold billions of dollars over decades for more accurate and timely information about the state of the world. Real estate developers, insurers, and energy producers could turn the data from that map into dollars.
But holy grails are, by definition, difficult to obtain. The map doesn’t currently exist, and it’s not for lack of trying.
Array Labs thinks that now is the time to build it. And its founder, Andrew Peterson, thinks he knows how.
The main thing you need is Very High Resolution Imagery (VHR) of gigantic swaths of Earth, freshly collected daily, to start, and more frequently over time. There are a few ways, theoretically, you could collect the data.
You could send out a fleet of LiDAR-equipped self-driving cars, with humans behind the wheel. That’s how Autonomous Vehicle (AV) companies do it today, one city at a time. But it’s too expensive and impractical to cover the globe. At $20 per mile, it would cost over $1 trillion to map the Earth’s surface, even assuming cars could drive everywhere.
You might fly LiDAR-toting airplanes across the globe. When people want VHR of specific areas today, that’s what they do. At around $500 per square kilometer (apologies for the mixed units, but we’re going with industry standards), a single US collection would be $5 billion. A global collection would run you a cool quarter-trillion, assuming you can access all of the world’s airspace without getting shot down. Closer, but still not good enough.
Let’s go even higher, from ground to planes to satellites. Satellites rarely get shot down. They scan the entire globe, airspace be damned. Once in orbit, they keep flying around and around and around at very high speeds. And they’re way cheaper: at current retail pricing of around $50/sq. km, it would only cost you $150 million to cover the US, or $7.7 billion to cover the whole Earth.
We’re getting warmer, but there are still two main problems with this approach:
Resolution. Today, the resolution from even the best 3D satellite collections is still 100x worse than airplanes. You couldn’t guide self-driving cars with these maps.
Coverage Cost. Getting coverage by sending up enough high-resolution satellites to capture the whole Earth would be too expensive to pencil out.
For a number of reasons we’ll get into, you can’t simply scale up current earth observation satellites to larger and larger sizes. They get exponentially less efficient as you scale them, and assuming you worked all of that out, a satellite big enough to provide the resolution and coverage you’d need would be way, way too big to send up on a rocket.
If you wanted to make the antenna really big – like 50 kilometers in diameter big – it would be impossible with anything close to our modern capabilities. Such an antenna would be 1,000x taller and 5,000x wider than SpaceX’s Starship. It would be 60x taller than the Burj Khalifa. It would have 5.82x the diameter of CERN’s Large Hadron Collider.
I tried to make an image to scale but it doesn’t come close to fitting on the screen. So let’s try a metaphor. If Starship were the size of a soda can, this 50 km diameter satellite antenna would be the size of the island of Manhattan.
But a diameter that size is useful, because it helps increase vertical resolution, a key piece if you want a true 3D map instead of a stack of high-resolution 2D pictures.
But having something that behaves like an enormous imaging satellite, or even just a giant radar antenna, would be really useful...
There’s a way to do that, but it’s kind of crazy.
What if instead, you kept the radar imaging satellites very small, but sent dozens of them up there in a big ring?
When you do that, what you end up with is something that behaves like an absolutely enormous antenna – an antenna 30 miles wide – that improves exponentially.
Instead of a monolithic antenna that gets exponentially less efficient with scale, you end up with a distributed system that gets more and more efficient with every satellite you add. Each satellite has its own solar panels, providing plenty of power. Each one is small and stackable enough that the whole cluster fits on a single port on an ESPA ring, snuggling in amongst all of the other satellites on the next SpaceX rideshare mission. Pointing them at the target is as easy as adjusting a small cubesat, like directing a ballet with dozens of prima ballerinas.
“Then, pretty soon,” Andrew explains, “You've got the most efficient image collection system that ever existed in the history of mankind.”
That’s what Array Labs is building, the crazy way: the most efficient image collection system that ever existed in the history of mankind. Its mission is to create a daily-refreshed, high res 3D map of Earth.
To be sure, Andrew’s idea for Array Labs is crazy in a lot of ways.
For one, the Air Force Research Lab tried to use radar to make a 3D map of the Earth back in the early 2000s with a program called TechSat-21. Working with DARPA, they designed the system to demonstrate formation-flying of radar satellites that would work together and operate as a single “virtual satellite.” It was an idea ahead of its time, sadly, and the program was scrapped due to significant cost overruns.
For another, earth observation isn’t necessarily the rising star of the space economy these days. There are already a bunch of EO satellites orbiting our Blue Marble. Incumbents and upstarts alike, companies like Maxar, Planet, and Blacksky, have a lot of capacity in orbit. A workable business model has been harder to come by. Planet and Blacksky are both trading near all-time lows after coming public via a SPAC in 2021.
When Ryan Duffy and Mo Islam wrote a piece on The Space Economy for Not Boring last year, they weren’t particularly bullish on the EO market, which was why I was surprised when Duffy called me a couple of months ago to tell me about a company I absolutely had to meet … in EO.
He’d gotten bullish – he was doing consulting work for them, and asked to take his pay in equity instead of cash – but after five years at media startups writing newsletters about emerging tech, he told me that he was planning on doing his own thing for the foreseeable future. So I was doubly surprised when, on our next call, Duffy told me that he was giving up the consulting life early to join Array Labs full-time as Head of Commercial BD.
After he introduced me to Andrew, I understood why.
Array Labs has many of the characteristics I love to see in a startup. It’s ambitious and just the right kind of crazy. It’s reanimating an idea that didn’t work before but might now thanks to exponential technology curves. It’s turning something traditionally monolithic into something distributed. It’s using technological innovation to unlock business model innovation, and executing against a clear strategy while it’s still in its uncertainty period. And, if it works, it will enable new businesses to be built that aren’t possible today.
In short, Array Labs both has its own powerful answer to the question, “Why now?” and the rare opportunity to serve as other companies’ answer.
But taking advantage of a powerful “Why now?” means that it’s also very early. Array Labs plans to launch four demonstration satellites next year and two more orbital pathfinder missions in 2025, before sending up its first full cluster in 2026. Risks abound.
That makes it a particularly fun time to write about the company. This essay is a time capsule from before Array Labs becomes obvious. It’s a Not Boring thesis paper of sorts, and the thesis is this: a company with a near-perfect why now (and the right team to capture it) and a strategy to match the moment can both disrupt and grow an established industry while enabling new ones.
To lay it out, we’ll need to cover a lot of ground in one pass:
Earth Observation: The 600km View
The Engineering Magic of SAR
How to Make the Most Efficient Image Collection System Ever
Intersecting Exponentials
Array Labs’ Strategy
Enabling New Markets
Risks
Mapping the Path to Mapping the World
Put on your spacesuit. Let’s launch into orbit to get a better view of the industry.
Earth Observation: The 600km View
A couple of weeks ago, I wrote, “Any technology that is sufficiently valuable in its ideal state will eventually reach that ideal state.”
The earth observation market is sufficiently valuable, even in its current state. In 2022, estimates put the market size around $7 billion and growing at roughly 10% annually. This is likely a fairly significant underestimate, as the US and other governments spend billions of dollars on highly classified programs.
But the earth observation market isn’t close to its ideal state. In its ideal state, earth observation would produce real-time, high-resolution, global coverage, easily consumable by the naked eye or machine learning models, accessible to anyone for their specific needs.
AV companies would ingest point clouds that turn into real-time maps, alerting cars of changes in traffic conditions, construction projects, or closed roads.
The Department of Defense would have real-time visibility into the movement of enemy resources, no matter the weather or time of day.
Environmental groups would be able to track planetary indicators by the minute.
Augmented Reality (AR) companies would design experiences that interact with the real world in real-time.
Foundation model companies, always hungry for more data to scale performance, could train their models on 3D and HD continental-level scans of the world.
If the data was good and cheap enough, entrepreneurs would certainly dream up uses that I can’t.
In its ideal state, earth observation will expand humanity’s ability to understand, respond to, and shape events on a global scale.
And we’re getting closer. Over the past century, we’ve made significantly more progress towards that ideal state than we did in all of the millennia of human history before it.
Here’s the progress in mapmaking over the two millennia between the first world map in the 6th century BCE and the first modern one in 1570:
No disrespect to the cartographers. Stitching together a map from ground level was an arduous, and even dangerous, proposition. But the maps were of dubious accuracy, nowhere near real-time, and certainly nowhere near close to real-time enough to fight a war with. So during World War I, governments experimented with a number of ways to get close-to-real-time images from above, including pigeons, balloons, and kites:
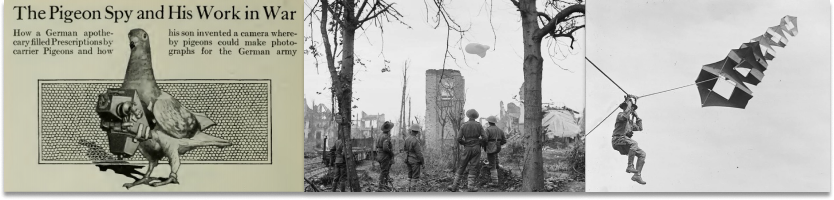
The war spurred government funding and public-private innovation, as wars tend to do. During World War I, they started putting cameras on planes, and by World War II, combatants on both sides sent planes higher and higher up to get better and better views, and to make them harder to shoot down.
World War II ended and the Cold War began, and both the US and the Soviet Union upped their spy game. To get a sense of the lengths the government was willing to go to to get aerial intelligence on the enemy, I highly recommend listening to the Acquired episode on Lockheed Martin, starting at around the 34 minute mark, where they cover the development of the U-2 spy plane and the launch of the Corona satellite program:
The U-2 plane that Lockheed’s Skunk Works developed flew 70,000 feet above the earth, higher than airplanes had ever flown before, so high that its pilots had to wear spacesuits. Edwin Land himself developed the camera that could take pictures from that high up.
The U-2 flew over the Soviet Union for the first time on July 4, 1956, and for nearly four years, the US was able to keep tabs on the enemy from above. Then, on May 1, 1960, the Soviets shot it down with a ground-to-air missile. We’d need to go higher: to space.
The Soviets famously launched the first satellite into orbit, Sputnik, in 1957, kicking off the space race. One of America’s biggest concerns was that with satellites, the Soviets would be able to collect better intelligence on us than we could on them. But it was the Americans who launched the first satellite capable of photographing the Earth from orbit: Corona.
The first Corona satellite went up in August 1960, and over the course of its month-long mission, “produced greater photo coverage of the Soviet Union than all of the previous U-2 flights combined.” Earth observation from satellites had begun in earnest.
Things were different then than they are today. The satellites housed film cameras with a then-impressive 5-foot resolution. But film? How’d we get the images back? Planes caught them in mid-air as they fell back to earth!
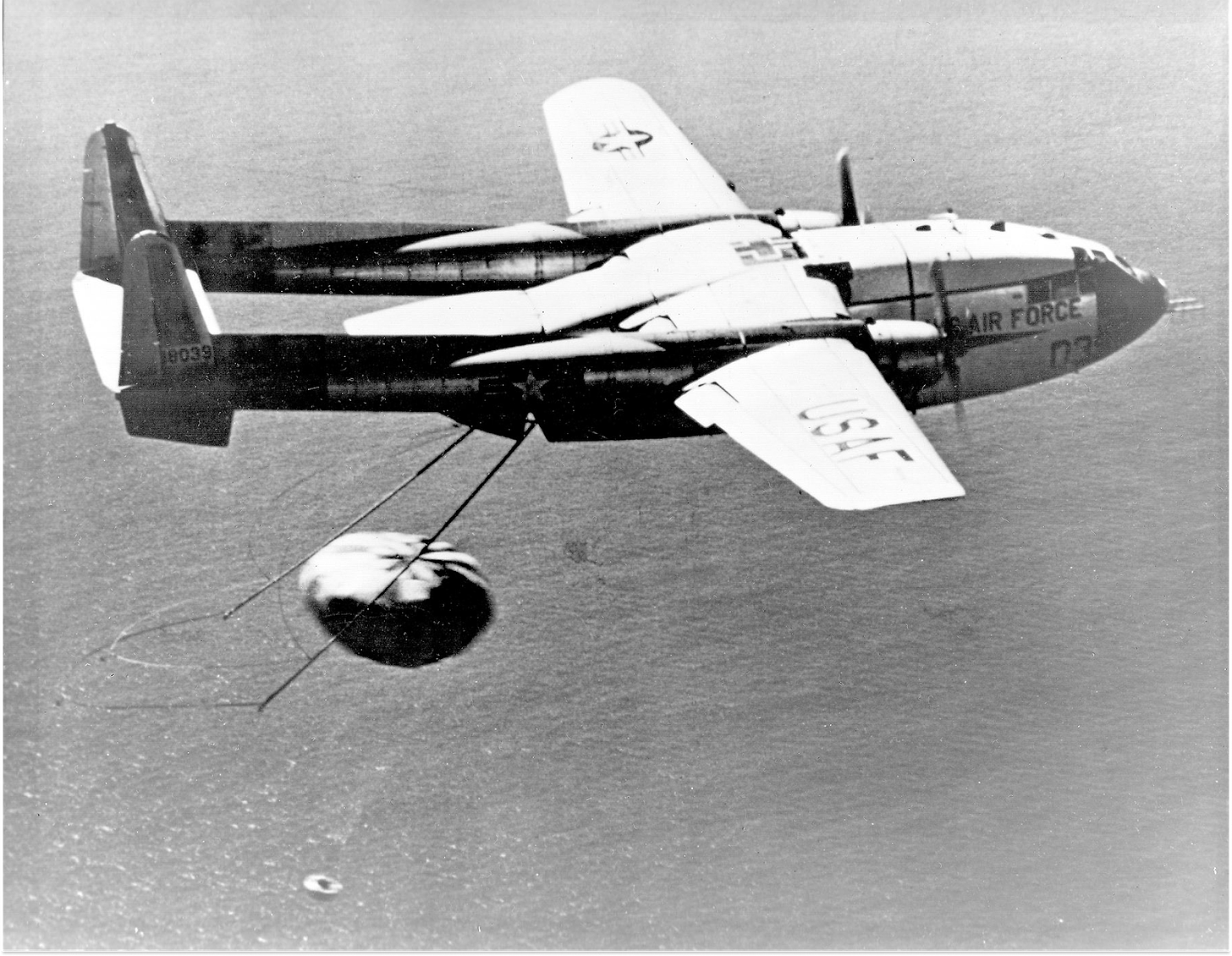
Satellite imaging technology has evolved a great deal since the 1960s. We now capture images with incredibly sophisticated cameras and beam them straight down to ground stations on Earth. I could nerd out on the history for pages and pages, but I have a piece to write, so if you want to go a little deeper, Maxine Lenormand made an excellent video covering the history and basic technical details of satellites:
For now, let’s fast-forward to today.
Today, the largest EO company, Maxar, which was taken private by a PE firm for $6.4 billion at the end of 2022, and the leading (publicly traded) startup, Planet, both primarily operate optical imaging satellites.
Optical imaging satellites capture photographs of the Earth's surface using visible and sometimes near-infrared light, like a traditional camera but in space. Given that these space cameras shoot from roughly 600 kilometers above the earth, continuously, the images they’re able to produce are mind-blowing.
Here’s an image from Planet’s Gallery showing flooding in New South Wales on November 23, 2022 in both visible (left) and near-infrared (right):
And here’s one from Maxar, showing an airport (on the site, you can hover over parts of the image to magnify them, like I did over the airplane):
We’ve come a long way from hand-drawn maps and pigeons.
While they both use optical imaging satellites, Maxar and Planet have taken vastly different approaches to the trade-off between resolution and coverage.
This is one of the key trade-offs, maybe the key trade-off, in EO.
Higher resolution allows for more detailed images but over a smaller area, while lower resolution can cover a larger area but with less detail. If you opt for very high resolution (VHR), you can overcome coverage by putting a lot of satellites on orbit, but VHR birds don’t come cheap. If you opt for high coverage but low resolution, there’s not much you can do to go higher resolution without launching new, more expensive satellites.
Maxar and Planet’s approaches set the edges of the trade-off space.
Maxar’s satellites are the highest-resolution commercially available, with a ground sampling distance (GSD) of 30 centimeters. A 30 cm resolution means that one pixel in each image represents a 30 cm by 30 cm square on the ground.
These satellites look like what you think of when you think of a satellite, small-SUV sized craft with solar panels, a big camera, thrusters, gyroscopes, and plenty of other bells and whistles.
Built mainly for the military, they aren’t really used to cover the whole earth regularly, but are tasked, meaning that a customer can say “go take a picture of this specific place” and Maxar will add the location to the target list to collect next time it’s in view.
In 2022, before Maxar went private, it reported that two-thirds of its Earth Intelligence revenue, $722 million, came from the US government. The government has budget and is willing to pay for capability, and Maxar delivers by delivering expensive, highly-capable satellites. The WorldView-4, which it launched in 2016, was produced by Lockheed Martin for a cost of $600 million. It suffered a gyroscope failure in 2019 and is no longer in orbit.
Maxar has announced its six-satellite WorldView Legion constellation, which is rumored to cost in the range of $100 million each and offer 25 centimeter resolution, and is scheduled to launch the first two with SpaceX on Halloween. Currently, Maxar has four satellites in orbit, as seen in this awesome real-time visual from Privateer’s Wayfinder (the fourth is somewhere near Australia in this shot):
But high-res optical satellites like Maxar’s run into the laws of physics when trying to scale up to even higher resolutions.
For VHR satellites, think about the high-res optical sensor as a tiny little soda straw. To snap those super-high-res 30 cm pics of the locations you care about (before you go whizzing by overhead and those places are out of view until tomorrow), you’re slamming and slewing that soda straw all over the place. Slamming means rapidly adjusting the satellite’s orientation to focus on a specific target, and slewing means rotating or pivoting the satellite to keep the camera aimed at the target while moving.
Now, the problem is that in order to make smaller pixels from the same altitude, you’ve got to increase the size of the satellite (specifically, the diameter and length of the telescope). This also tends to reduce the field of view. This means that as your pixels get smaller, your straw gets narrower, and it also gets much, much harder to move. (In fact, doubling the resolution of your satellite makes it roughly 40 times more difficult to slew.)
To perform these feats of agility, big satellites make use of larger and larger Control Moment Gyros (CMG’s), exquisite, high-speed gyroscopes which are pushed and pulled against in order to rapidly slam and slew the little soda straw back and forth, from place to place, when flying over a particular area. That way, they can ideally capture everything on their tasking scorecard.
It’s no surprise just how expensive spy satellites can get. In the 2000s, as the US looked to put two new “exquisite-class” satellites, US Senator Kit Bond said that the initial budget estimate for the two KH-11 sats was more than it cost to build the latest Nimitz-class aircraft carrier!!! The two satellites would end up being produced two years ahead of schedule and $2 billion under budget, for a total of $5.72 billion in today’s dollars. So, less than an aircraft carrier, and a W, in terms of timeline and budget, but not cheap.
The long and short of it is that high resolution optical imaging can get really expensive, and that the higher resolution you go, the worse coverage you get.
Planet takes a different approach, optimizing for coverage. The company was founded in 2010 by three NASA engineers who realized that iPhone cameras had gotten so good that they could put off-the-shelf cameras on off-the shelf cubesats (very small satellites) to create cheap optical imaging satellites. Instead of one really high-resolution $600 million satellite, they could throw hundreds of pretty low-resolution satellites into orbit, each less than $100k, collect an image of the entire planet every day at 3-5 meter resolution, and sell access to the images as a subscription.
Instead of selling to just the military, Planet, which established itself as a Public Benefit Corporation, would heavily focus on serving commercial customers, such as those studying climate change, who need frequent revisits to measure change, but aren’t as sensitive about resolution. Planet’s Doves aren’t taskable – you get whatever they fly over, and they make it up by trying to fly over more areas with higher coverage.
In 2017, Planet acquired the satellite company Skybox from Alphabet just three years after Alphabet bought it for $500 million. Skybox’s SkySat sits somewhere between Maxar’s WorldView and Planet’s Dove. They’re about the size of a minifridge, cost around $10 million, and deliver 50-70 centimeter resolution. Planet has announced its next constellation, Pelican, which will replace SkySats. It’s aiming for 30 satellites at about $5 million each, which will offer 30 centimeter resolution, up to 30 captures per day, and a 30-minute revisit time.
According to Wayfinder, 362 Doves and 40 SkySats have been launched.
There’s a lot more detail to dive into on Maxar and Planet – I found this Tegus interview with Maxar’s CEO to be an excellent guide – but for our purposes, the point is just to show the two ends of the spectrum in optical imaging satellites: high resolution vs. high coverage.
But optical imaging satellites aren’t the only way to snap high-resolution images from the heavens.
In the YouTube video I shared earlier, Maxine introduces a different approach by saying, “Every once in a while, humanity invents some weird, delightfully clever piece of engineering magic, and then gives it a convoluted name. This is one of those.”
That convoluted name is Synthetic Aperture Radar, or SAR.
The Engineering Magic of SAR
SAR achieves high-resolution imaging by simulating a long aperture by attaching a radar to a moving object, like a plane or satellite. The aperture effectively becomes as long as the section of the flight path during which the radars are aimed at a given target.
If you want to understand why SAR is important, and what makes Array tick, this is the key formula you need to grok:
Image quality = Aperture Size / Wavelength
Remember when I was talking about higher resolution spy satellites needing to be larger? This is the formula that explains it. If you’ve seen the lenses that professional photographers use, you’ve seen that larger diameter lenses take better photos (or at least, higher resolution photos).
What you might not know is that the same formula works for every type of imaging.
That extreme-UV lithography for etching those silicon chips? That’s smaller wavelengths making things better. Radar imaging is also subject to this formula.
Radar has a number of advantages over optical imaging:
All-Weather Capability: radar penetrates clouds, fog, and rain
Day and Night Visibility: radar systems can operate in complete darkness
Penetration: radar can see under tree cover and even below soil or shallow water
Different Information: radar can pick up roughness and surface material
Coherent Integration: radar pulses can be added together for increased power and resolution
But it also comes with its own challenges, chief among them that radio waves are so long.
Optical imaging is called optical because it uses visible light, which has wavelengths of 400-700 nm. Radar uses radio waves, which have 1 mm to 1 km wavelengths, or ~10,000x as long as visible light’s.
Plugging radio waves into our image quality formula, we get a denominator for radar that’s 10,000 times bigger than that of optical imaging. That leaves two choices, if you insist on using radar because those advantages are worth it to you:
Accept a 10,000x lower-resolution image
Make your aperture 10,000x bigger
If you want to compete in the earth observation or aerial imaging (same thing but with planes) markets, 10,000x worse image quality isn’t going to cut it. But as we discussed earlier, making an aperture 1,000x or 10,000x larger than a camera’s means that you can’t fit it on a rocket (or a plane). Hmmmmm 🤔
Luckily, there are geniuses in this world, and in the 1950s, two sets of them – engineers at Goodyear Aircraft Corp. in the US and at Ericsson in Sweden – struck upon a similar idea: what if we move the radar antenna over the target, by flying it on a plane, fire radio waves pulses the whole time, and capture the signals as they bounce back up?
Turns out, doing that allows you to create something that behaves like a much larger aperture synthetically, hence synthetic aperture radar. A 10 km flight path essentially gives you a 10 km aperture. SAR along a flight path gives you higher azimuth resolution, essentially increasing the horizontal sharpness of side-by-side items in the image. Vertical sharpness, or range resolution, is created by essentially measuring radar round-trip-time to determine how far away certain objects are.
The US government, and particularly the defense and intelligence agencies, were very interested in a technology that could penetrate clouds and trees, and by the late 1960s, had developed SAR systems with one foot resolution. In the 1970s, the National Oceanic and Atmospheric Administration (NOAA) and NASA’s Jet Propulsion Lab (JPL) launched the first SAR satellite, Seasat, to demonstrate the feasibility of monitoring the ocean by satellite.
Until very recently, space-based SAR was the domain of wealthy governments who could afford the estimated $500 million to $1 billion price tag per satellite that the National Reconnaissance Office (NRO) ponied up for its Lacrosse Satellites. These things were expensive in large part because they were massive. Look how small they make these two humans look:
In recent years, thanks to decreasing launch costs and technological advances, startups have gotten into the game. The three most impressive include Umbra, Iceye, and Capella. Here’s a quick overview of their capabilities:
In August, Umbra dropped the highest-resolution commercial satellite image ever at 16 cm resolution. The only higher-resolution image the public has seen is this (likely classified*) 10 cm resolution image former President Trump tweeted in 2019 of the Semnan Launch Site One in Iran.
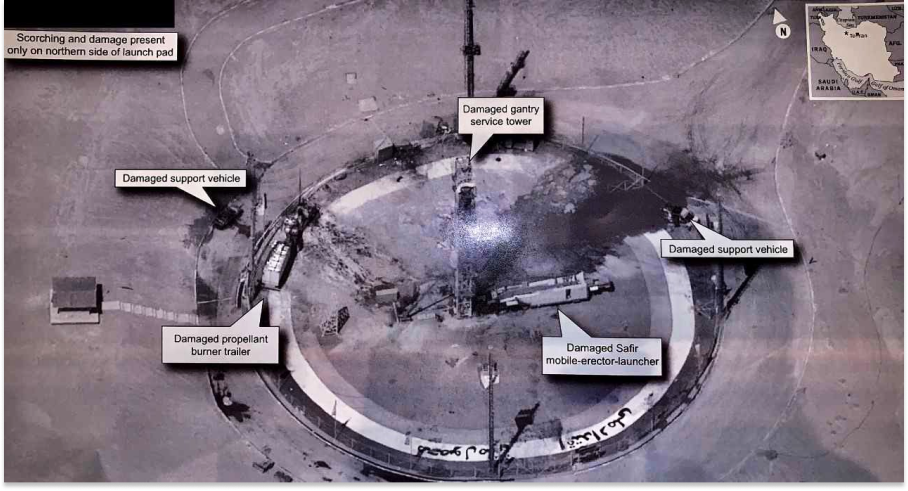
At the time, the image revealed that US spy satellites were 3x better than the best commercially available imagery. Now, that delta is shrinking. Another Y Combinator space startup, Albedo, aims to capture 10 cm optical imagery by flying its satellites very low.
OK. Let’s return from the espionage-laced optical detour back to SAR.
One thing to note about the 2D SAR satellites is that they have two main modes: spotlight and stripmap. Spotlight mode allows them to focus radar beams on specific areas of interest at higher resolutions, while stripmap mode allows them to monitor larger areas at lower resolutions (think about it like the slew/slam soda straw dichotomy mentioned earlier).
Commercial SAR satellites can switch between spotlight and stripmap—and turn the dial between resolution and coverage. It’s a step closer to eliminating the trade-off between resolution and coverage.
But if we’re looking for a 3D map of the whole Earth, we need both the resolution and coverage turned up at the same time, and we need the images to be 3D.
Turns out, you can make a 3D image from 2D satellite data. How?
First, you take a stack of satellite images of the same location, but taken from different angles. Then, you perform a process called 3D reconstruction, where you analyze how different groups of pixels move relative to each other in each of the images. Combining that information with the knowledge of where the images were taken allows the 3D information to be extracted. Up to a certain point, the more images used, the higher the quality of the resulting 3D reconstruction.
Unfortunately, it’s pretty hard to obtain a better 3D reconstruction than the 2D data you started with, and you often need to wait for a long time to gather all of the different angles you need in order to perform the 3D reconstruction. You run into the resolution vs. coverage trade-off in another dimension.
Is there a way to improve 3D image quality and get all of the data we need in a single pass?
That’s what Array is cooking up.
How to Make the Most Efficient Image Collection System Ever
Andrew Peterson is an aerospace engineer who’s worked in radar for a long time, first at General Atomics, where he worked on “Design and implementation of advanced SAR image formation and exploitation techniques, including multistatic and high-framerate (video) SAR” and “Guidance, navigation, and control systems for hypersonic railgun-launched guided projectiles and anti-ICBM laser weapons systems,” and then at Moog Space and Defense Group, where he “Worked on beam control systems for the world's largest telescope (LSST).”
(I, for comparison, write a newsletter.)
When we first spoke in July, the first thing he told me was: “I was happy being a technical guy forever. I never thought I’d start a company. I still don’t introduce myself as an entrepreneur. But then I had an idea that was way too good.”
Right around the time he had the way-too-good idea, a coworker gifted Andrew two books: one was about cows, and one was Peter Thiel’s Zero to One: Notes on Startups, or How to Build the Future. Fortunately, he mooooooved right past the cow book and dug into Zero to One.
In the book, Thiel presents his now-famous question: “What important truth do very few people agree with you on?”
Most of us get stumped by this one, think of something controversial that a lot of people do agree with you on, or resort to hyperbole to outdo others who only kind of agree. Andrew, after years in aerospace and radar, had a very specific, technical answer. That answer was the intro to this piece: you can build the world’s most efficient 3D image collection system by putting a cluster of simple radar cubesats into a giant ring.
He believed that swarms of dozens of satellites (just how many, I can’t say, because I’ve been sworn to secrecy) working together, orchestrated by software, could outperform the monolithic satellites that the rest of the industry was working on.
“If you think about scaling, as you continue to scale up an antenna to be larger and larger and larger, it gets exponentially less efficient. You cannot scale these things,” Andrew says. “But what if you recast the problem as scaling distributed systems?”
I’ve been listening to the latest Acquired episode on Nvidia & AI, so I’ll go ahead and make the analogy: if traditional EO satellites are CPUs, what if you built the GPUs?
In the world of computing, CPUs hit a wall in the early 2000s when it came to clock speed and power consumption. By 2005, Dennard scaling, which allowed for performance gains without increased power consumption as transistors got smaller, broke down. Clock speed, the rate at which a CPU or other digital component executes instructions, measured in hertz (or more frequently megahertz or gigahertz), leveled off. Pundits proclaimed Moore’s Law dead, as they’re wont to do. The very next year, Intel dropped an elegant solution: the Pentium Dual-Processor. Doubling the cores required interconnects to allow them to communicate and coordinate tasks, but it worked. Moore’s Law lived on.
Modern high-end desktop and server CPUs can have up to 64 cores, but there are diminishing returns and CPUs with more cores eat a lot of power.
GPUs, on the other hand, were designed from the ground up to be parallelized. With thousands of smaller, more specialized cores, they’re built explicitly to handle processing lots and lots of data at once, much more efficiently than CPUs. While Jensen Huang and Nvidia created GPUs to handle graphics, it turns out they’re perfect for training AI models. If Moore’s Law ever dies, Huang’s Law will be there to take the baton.
Array Labs is taking a similar approach to satellites, designing a system of satellites from the ground up to be parallelized.
Traditional EO satellites are like the CPUs: powerful but limited in how much they can scale. They're big, expensive, and can only be in one place at one time. You can send more of them up, but like adding CPU cores, there are diminishing returns if you’re looking to build a 3D map.
Array Labs, on the other hand, would deploy clusters of smaller, cheaper satellites that can work together in tandem, much like the cores in a GPU.
This configuration is called multistatic SAR – instead of a single antenna sending and receiving signals, multiple antennas send signals down and each receives the signals from all of the other antennas in the cluster. So if you have a dozen satellites in a cluster, each submits a pulse and all receive twelve pulses, for a total of 144 image pairs of a particular scene.
The upshot is that, once you stitch it all together, you can create better images, with both better resolution and coverage.
As an example, in April, Capella ran a test by turning two of their satellites into a bistatic SAR (same idea, but with just two SAR satellites instead of dozens), and the difference in image quality is stark:
Capella’s test was basically a higher-resolution spotlight mode shot, but Germany’s TanDEM-X has been flying bistatic SAR since 2010 for scientific research, and this artist’s rendition shows another major benefit of even bistatic SAR: it can cover wide swaths in each path (coverage).
Andrew had a bigger idea. Instead of spending €165 million for two satellites, like Germany and Airbus did on Tandem-X, what if he could spend single-digit millions for dozens of cubesats with high-quality, miniaturized, components? Array’s "swarm" approach would enable both higher resolution – up to 10 cm – and better coverage – swaths over 100 km wide in each pass.
All of that was the theory, at least. And then Andrew ran the numbers, and on paper, it worked.
If you double the number of satellites in a cluster, you double the aperture size (and therefore the image quality) and the amount of antenna area you have in space.
That halves the transmit problem: you need half as much power to transmit down to the ground.
Plus, you have twice as many solar panels up there collecting power.
The upshot is, when you double the number of satellites, you quadruple the daily collection rate – the amount of data that can be captured or transmitted – for just double the cost.
Instead of working against you, scaling works for you. The more satellites you put in the ring, the better it performs (once you write a lot of complex software and get them to work together).
Adding a height dimension, say with a resolution of 5 cm, multiplies the number of pixels Array can collect on any object. The more pixels you have, the better the computer inference (aka, AI) gets. So in a funny coincidence, Array’s data might be the key to delivering the high-fidelity 3D point cloud data needed to train AI models on the earth, just like the GPU was key to training large AI models in the first place.
On paper, 3D SAR is superior to both optical imaging and 2D SAR on every dimension that matters for building a high-refresh rate 3D map of Earth. He had to put the theory to the test.
So reluctantly, Andrew let his crazy good idea beat his conservative, technical tendencies, and in the fall of 2021, teamed up with Isaac Robledo to found Array Labs.
A few months in, in the summer of 2022, Array was accepted into YCombinator’s S22 batch. The vaunted accelerator has a simple motto: “Make something people want.”
That was another beautiful aspect of Andrew’s crazy good idea: he knew customers would want it, if he could pull it off. And he was pretty confident he could pull it off; it’s just physics and engineering.
The US government alone happily spends billions of dollars on related capabilities, and Array should be able to deliver those capabilities much more cheaply. On paper, it could deliver a combination of 10x better persistence and 10x better quality than optical imaging, at super low costs over very large areas, refreshed frequently at scale.
Privacy Pause: It’s important to note that while Array’s data quality should be excellent, it won’t be facial-recognition excellent. It won’t be able to identify people or license plate numbers, and it won’t see colors. So its existence shouldn’t pose concerns about individuals’ privacy.
Not only would Array make something that the government would want, it could flip the tasking model on its head and sell anyone a subscription to the same dataset (maybe without access to the highest-resolution images over Area 51). On paper.
But they still had to build the thing, and that meant putting together a team.
Array is headquartered, peculiarly enough, in Palo Alto, the heart of Silicon Valley.
Why is that peculiar? Because, while the Silicon Valley ethos may dominate mindshare of New Space companies, relatively few are actually based there. SpaceX, Hadrian, and Varda, for example, are all in or near LA.
Remember the Corona program I mentioned earlier? Well, turns out in the late ‘50s to ‘60s, the CORONA birds were covertly produced for the CIA in the Palo Alto plant of the Hiller Helicopter Corporation. In that sense, Array Labs’ Palo Alto roots are a full-circle moment for the Valley. The US government arguably seeded Silicon Valley, buying from companies that built semiconductors for aerospace and defense programs. As Silicon Valley came of age, though, it moved away from the government. Now, we’re so back.
Array set up shop in the Valley not for that nostalgia factor, though, nor to be near the VC dollars on Sand Hill Road, but so that it could recruit 99.99th percentile software and hardware engineers, the kind of bare metal bandits with experience at the Qualcomms, Amazons, and Metas of the world. (If that sounds like you, Array is hiring.)
The brains and hands Array has … clustered … in its Palo Alto HQ have not only worked on railguns, or helped develop a 26-foot fully robotic restaurant and the most powerful Earth-observing satellite ever, they’ve also led teams designing silicon, shipping semiconductors, laying the groundwork for mass-market augmented reality contact lenses and headsets, and more. They hold patents and have developed IP for technology that can be found in more than 100 million modems worldwide. That’s the kind of volume production and scale that aerospace engineers could only dream of.
Space is sexy, sure, but Array lives and dies by systems and software that are already ubiquitous here on Earth. Satellites, of course, but also semiconductors, wireless communications, digital signal processing, and RF (radiofrequency)/analog design. The team is a collection of experts in each piece of technology on which Array relies.
Economies of scale exist for the billions of components that are miniaturized and integrated into smartphones every year. Array Labs is riding the miniaturization wave like others who have come before it, but also bandwagoning on the proliferation of 5G. Technologies like multiple-input and multiple-output RF links, developed for next-gen wireless networks here on Earth, can also be put to work in distributed space swarms.
As Varda CEO Will Bruey put it, “Each piece of what Varda does has been proven to work; what we’re building is only novel in the aggregate.” The same could be said for Array.
It’s weaving together a number of curves that have hit the right place to build the most efficient image collection system ever, at the right time.
Intersecting Exponentials
The Array cluster is going to look something like this when it goes up in 2026, give or take a few cubesats:
That doesn’t look like any of the monolithic satellites I’ve shown you so far. It’s a distributed swarm of cubesats operating as one very large, very powerful, and still very cheap satellite; a fresh take on a crowded industry.
In I, Exponential, I wrote that, “Many of the best startup ideas can be found in previously overhyped industries. Ideas that were once too early eventually become well-timed.” That applies here.
Earth observation is one of the few pieces of the space economy that isn’t practically as empty as space itself. Governments, defense primes, incumbents, and startups alike dot the landscape. Some have been successful, more have been expensive science projects.
So the big question for Array is: why now?
In one of 19 frameworks in a Google Doc titled Frameworks v0.2, Pace Capital’s Chris Paik explains why it’s important for startups to have a compelling answer to the question, “Why Now?”:
Venture capital is particularly well suited to finance companies that are capitalizing on ‘dam-breaking’ moments—sudden changes in technology and regulation (and to a lesser extent, capital markets and societal shifts).
I’ve come across very few companies, maybe zero, with a more comprehensive “Why now?” than Array.
Array Labs’ “Why Now?” is a mix of commercial, geopolitical, and technological factors.
On the commercial side, there has never been greater demand for 3D point clouds of the Earth. For one thing, with the rise of AI, we now have models capable of doing something with all of that data, and so much economic activity in the space that both the models’ capabilities and thirst for fresh data seem likely to continue to grow.
As one specific application, Autonomous Vehicle (AV) companies are actually making money now by offering robotaxis in select cities. In order to expand to new markets, reliably and affordably, they need better and cheaper 3D maps.
On the geopolitical side, the war in Ukraine and growing tensions with China mean that the US government has an even greater appetite for real-time, high-resolution images of the earth than normal. Last year, it spent roughly $722 million with Maxar alone. It also represents 50% of Umbra’s revenue, according to TechCrunch, and its allies represent an additional 25%. The government is a logical early customer, and non-dilutive funder, of Array’s product.
There are no guarantees in startups, but if Array Labs is able to deliver on the product it plans to build, the demand will be there. Customers like the combination of cheaper and better.
So can they build it? Array is taking the non-differentiating components – the cubesat, the launch – off the shelf. It’s building bespoke radar, antenna, and algorithms – the things that will make or break the company – with its “Blue Team” of experts who have built similar things before.
On both the buy and build side, there are strong technological tailwinds, including:
Cheap Launch Costs
Cheap, Performant Cubesats
Modern Telecom
Cheaper, More Compact Memory Systems
ESPA Rings and Flatstacking
Cheap Launch Costs. It would not be a space essay if I didn’t whip out the chart.
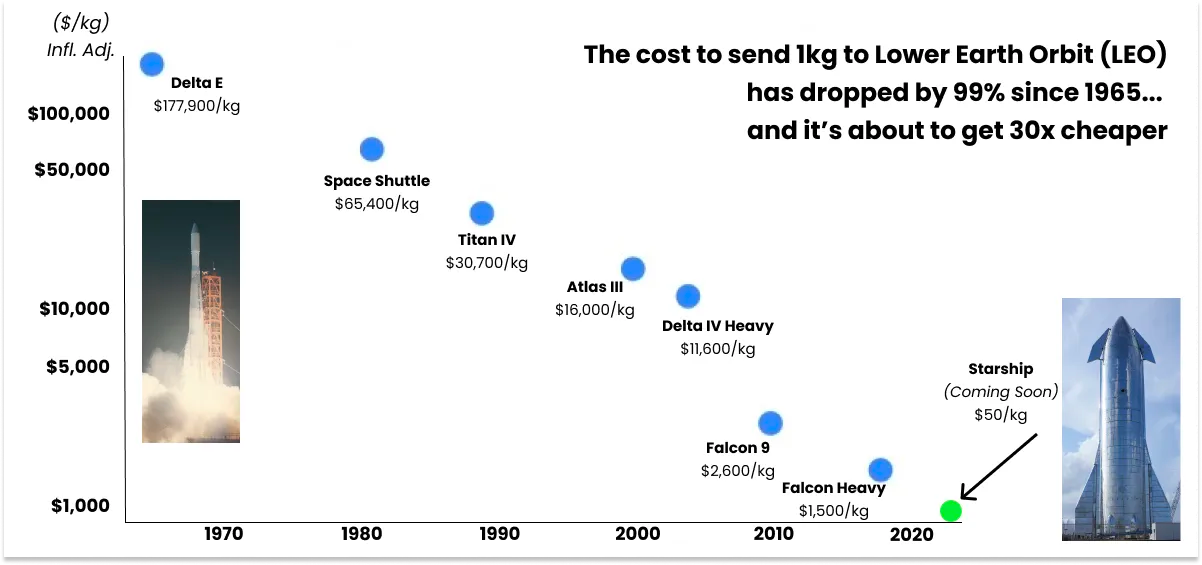
The cost of sending a kilogram to LEO, where Array will put its clusters, has fallen by two orders of magnitude since the 1970s. Theoretically, SpaceX can send each kilogram up for $1,500, but since it’s the only game in town, it charges a healthy margin.
The fun thing is, you can actually plug in some Array numbers – first cluster’s estimated dry mass (150kg), orbital destination (sun synchronous orbit (SSO) / 550 km above Earth), and launch date (Q4 2025) – on SpaceX’s Transporter rideshare calculator, and get an estimated price: $830k, or $5,533/kg.
Cheap, Performant Cubesats. Thanks to the burgeoning space economy, you can now take all but your most differentiated parts off the shelf.
Cubesats, first launched by Cal Poly and Stanford in 2003, have come down in price by an order of magnitude over the past couple of decades, from ~$1-2 million to ~$100k today. Dozens of them now cost the same as, or less than, one larger, custom-built satellite.
Thanks to Moore’s Law and the miniaturization of electronic components more broadly, these small cubesats can still pack a punch. Think “the iPhone in your pocket is more powerful than the computers we used to put a man on the Moon,” but for satellites.
Modern Telecom. While the last two factors – launch and cubesats – benefit many companies, the learning curves in cellular and wireless telecommunications are more specifically beneficial to Array.
Array’s satellite is essentially an off-the-shelf cubesat with a 5G base station built in to send and receive the radar pulses. Thanks to the rapid buildout of advanced RF technologies across the globe, and the billions of dollars companies are pouring into it, telecom is getting exponentially cheaper.
Cheaper, More Compact Memory Systems. A constantly-updating 3D point cloud of the Earth captures a ton of data, which needs to be stored and transmitted.
In an interview with TechCrunch, Andrew pointed out that, “The system that they [TechSat-21] had come up with was ten spinning hard drives that are all rated together,” he said. “It weighed maybe 20 pounds, took 150 watts [of power]. Now, something the size of my thumbnail has 100 times more performance and 100 times less cost.”
ESPA Rings and Flat Packing. Smaller, cheaper 5G base stations and memory systems mean that Array can fit better capabilities than a bus-sized, hundred-million dollar SAR satellite from a couple of decades ago on a cubesat.
If you look closely at the satellites in the video at the beginning of this section, they look a bit like SpaceX’s Starlink satellites. That’s not a coincidence.
SpaceX wants to send a lot of satellites into orbit – there are over 4,500 orbiting earth today, with a goal of 42,000 over the next few years. To launch them cost effectively, SpaceX needs to be able to send a lot of them up at once. Part of that equation is building bigger rockets – like Starship – and part is fitting more satellites into the same space. SpaceX designed Starlinks to stack as tightly as possible inside the nose cone of the Falcon rocket. It can fit 60.
Array Labs designed its own satellites such that a whole cluster fits on a single one ESPA ring port, which was created by Andrew’s alma mater, Moog, and is used by SpaceX (and others) to launch secondary payloads and deploy them into orbit on its Transporter rideshare missions.
There are other advances Array is taking advantage of: DAC/s, ADC/s and FPGAs (RF chips) are getting faster and cheaper, more ground stations are popping up worldwide, and elastic compute and AI inference are moving at warp-speed, just to name a few. As demands from other industries bring components down the cost curve, and up the performance curve, Array can benefit. It’s how they’ve designed the system, drawing inspiration from SpaceX and others.
It’s not a technology curve, per se, but SpaceX’s example is another contributor to Array’s “Why Now?”.
In a 2021 interview, Elon Musk spelled out his five principles for design and manufacturing:
Make requirements less dumb
Delete part of the process
Simplify or optimize
Accelerate cycle time
Automate (but not too early)
These principles show up everywhere at Array.
Take Array’s formation flying. How do you get dozens of satellites to form, and stay in, a ring as they hurtle around the globe at 4 miles per second? Instead of adding thrusters, making them way more expensive, heavier, more complex, and less stackable, Array uses a novel ‘aerodynamic surfing’ approach, made possible in part by founding engineer and employee number 2, Max Perham, a former Maxar WorldView engineer and cofounder of Mezli, which allows them to fly through space without a propulsion system. Make requirements less dumb. Simplify.
Or the rigid antennas Array uses. They don’t deploy, they don’t look like radar antennas, they’re not disc-shaped. They’re just the most straightforward path to maximum antenna area at minimum cost.
If you wanted to build something with similar capabilities to Array’s cluster a decade or two ago, it would have been possible, just prohibitively complex, expensive, and slow, as TechSat-21 demonstrated. The technological curves Array is operating in the middle of just make it economically feasible. The company’s approach is all about building for scale, building cost-efficiently, and building fast.
Speed shows up everywhere at Array. In April, Array got the “dumb metal,” the structural components without the active, intelligent, or electronic functions, for its first test rig. Within a few months, the team had turned it into a fully functioning multistatic radar test range, building a robotic, rail-mounted SAR in the process, and used it to develop 3D imagery.
They went from the top left rendering to the bottom-right reality in roughly 100 days:
The successful test buys the team the confidence that the algorithms on the satellite will work. That’s critical, because while we’ve focused on the hardware, what Array is building is as much a software challenge as anything. SAR satellites have been around for a long time. It’s getting them to work together, to behave like one 50 km-wide satellite, to turn hundreds of image pairs into clear pictures and 3D point clouds customers can use. That's the magic, and the algorithms are the spell.
Andrew explained: “The test really burns down a lot of the technical risk.” With the test rig validation and algorithm experiments in hand, Array is completing its Preliminary Design Review (PDR) this Friday, a key step on the path to launch, less than one year after closing its seed round in November 2022.
Array Labs is moving so fast because Andrew understands the need to get the best product to market and change the industry’s business model before everyone else realizes that his idea isn’t as crazy as it sounds.
Array Labs’ Strategy
My second conversation with Andrew took place on July 20th, two days after I published How to Dig a Moat. When we hopped on the phone, it was the first thing he wanted to discuss.
Companies can be in the right place at the right time, ride the highest-sloped technology curves, with the right team, get to market first, and then watch all of their margin get competed away. Hardware innovations are notoriously difficult to protect from the erosive forces of competition.
Andrew realized that the company had a head-start, and an uncertainty window to play in before competitors woke up to the beauty of Array’s approach. Which moats could the team start digging?
In the very near-term, there are three.
First, he pointed out, there aren’t many radar people with SpaceX-like training. There’s a frenzy for SpaceX-trained talent, but RF engineers are an undervalued resource in the aerospace world. Array’s first order of business was to hire as many of the best RF engineers it could find and get them working like a well-oiled, fast-moving startup.
“Our hiring strategy is simple,” Andrew said. “Find uncommonly talented people and pay them uncommonly well.”
Locking in the best talent before other companies catch on could extend the lead a bit: if the best people were at Array, it would be hard for anyone else to match their speed and skill. But that’s not a durable moat.
Second, they could offer a product that’s both 100x cheaper than airplanes and 100x better quality than 3D satellites. Aerial data at satellite prices. That’s what they plan to do, and early revenue will fuel growth, speed up deployment, and extend the lead, but pricing isn’t a durable advantage.
Third, they could counter-position against the incumbents and startups in the space. If companies like Maxar, Umbra, and Iceye built their teams, business models, and products based on the idea that they needed to pack more performance into each individual satellite, Array could design simple satellites that were cheaper, easier, and faster to manufacture. To match Array, competitors would need to scrap their work or try to string together clusters of more expensive satellites. But counter-positioning, too, only buys you more time.
The master plan, though, is to turn the team’s technical innovation into a business model innovation.
The EO market, Andrew explained, suffers from the “tyranny of tasking.”
“Imagine you have a mine where you’re digging minerals, and there’s a forest around it. Two groups are interested in looking at that region: the mine’s owners/investors and an ESG company that wants to understand its environmental impact — which has the ability to pay more?”
The answer is typically the mine’s owners and investors, and so they’ll pay more to “task” the satellite to take higher-quality images. The EO company will sell the ESG company older, shittier images for a lower price.
Andrew thinks this is stupid. The image is there and the marginal cost to serve it is approximately zero. He thinks the business model needs to change.
Because Array Labs will be able to take high-res, near-real time images over very wide swaths, it doesn’t need to be tasked. Its first cluster will provide coverage of the 5% of Earth that houses 95% of people, hoovering up high-quality images the whole time—and refreshing every 10 days. Then, anyone who wants to access the data can pay a subscription to access it. Subscription models aren’t new – Planet and others offer subscriptions – but subscriptions on aerial-quality data at satellite prices are.
Because of Array’s low costs, it’s feasible that one large customer paying much less than they do to a competitor today could cover all of Array’s COGS on a cluster for a year. But since Array essentially captures images of everything (there’s no tasking) and downlinks the image data to an AWS S3 bucket, it can sell access to any number of companies at de minimis marginal cost.
Very roughly, the second customer would mean 50% gross margins, the third would mean 66.67% gross margins. By the tenth customer, you’re at 90% gross margins… on a business that builds radar satellites. It looks a lot more like a software business.
But even strong gross margins aren’t a moat. If anything, they’re a bright neon sign inviting competitors to come take some away. The first moat Array is looking to dig is scale economies.
Strong margins on healthy revenue should allow Array to deploy more clusters into orbit more quickly. As it launches more clusters, it will be able to offer an increasingly great product (more frequent refreshes, more coverage area). Almost like Netflix, as it acquires more subscription customers, it will be able to amortize its costs of content – high-quality images – over more subscribers. This makes the subscription more valuable to each customer.
So actually, the chart of gross margins might look something like this, with little dips as Array sends new clusters up. Each time, the dip is a little smaller, as the cost of each new cluster is amortized over more subscribers.
It should cost Array less to serve a better product than any new entrant. Scale economies.
But the way Array sees it, there are actually two types of potential customers:
Those who can mainline a constantly-updating stream of 3D point cloud data and do something with it themselves.
Those who can’t.
AV companies, the Department of Defense, and large tech companies like Google, Meta, and Nvidia have the tools and the teams to plug into an API, pull the data, and work their own magic on it. They can pay Array directly.
Others, like real estate developers, insurance companies, environmental groups, researchers, small AR developers, and more, should be able to find a use for the data, but they all have industry-specific needs, requirements, and sales cycles.
For this group, the company envisions operating more like a platform on top of which new companies might form to serve specific markets. End users in these markets might need algorithms to help make sense of the data, vertical-specific SaaS that incorporates it, or self-serve analytics tools which third-party developers can provide.
I might, as an example, form 3D Real Estate Data, Inc., pay Array for a subscription to the pixels, and use it to create a real estate-specific data product that I can sell to developers. At the end of the sale, I pay Array a 10% cut. That way, Array’s pixels – which are non-rivalrous – can impact dozens of industries without Array needing to Frankenstein its product and build out a huge sales force to serve those industries.
If it pulls that off, it has the potential to capture two powers, depending on the choices it makes.
The less likely is that it could provide data and resale licenses to third-party developers who build inside of an Array ecosystem, like an App Store for 3D Maps. Developers would build on Array, and customers would come to Array to find the products that fit their needs. In that case, it could be protected by something that looks a lot like platform network effects.
The more likely is that Array sells the data and licenses to third-party developers who are free to build whatever type of product they want and sell it wherever and however they can. Array would develop tools to make it as easy as possible for developers to build products with its data and would continue to grow the data set and increase the refresh rate as it adds more clusters. In this case, the switching costs to companies who’ve chosen to build with Array would be high.
Array plans to go to market with the self-serve model – serving AV, DoD, FAANG, and the like, at launch, and build out the platform as it grows. In both cases, it may be the “Why Now?” for a wide range of existing and yet-to-be-imagined companies.
Enabling New Markets
In Paik’s Frameworks, he writes of Being the ‘Why Now?’ for Other Companies:
If a company can deliver, mostly through technological innovation, an answer to the question, “Why Now?” for other companies, it will be a venture-scale outcome, assuming proper business model—product fit. The challenging part here is that the vast majority of the customer base for the innovating company does not yet exist at the time of founding.
To me, this is one of the most compelling aspects of Array’s business: it has the potential to be the “Why Now?” for other companies without the market risk.
Ideally, at some point, a number of previously-impossible companies will come to life thanks to the existence of a real-time 3D map of the world. Maybe they’ll be augmented reality companies that design experiences based on live conditions. Maybe they’ll be gaming companies that update their maps in real-time in response to the real-world. Maybe they’ll be generative AI companies whose image quality dramatically improves by training on so much Earth image data. More likely, they’ll be things I can’t imagine.
To bank on those companies' existence and success, however, would be a very risky bet.
In the meantime, Array has an established commercial ecosystem to sell into.
Airborne LiDAR is an industry that already generates $1B+ in annual recurring revenue. Airborne LiDAR’s TAM is gated by accessibility and survivability (you can't, for example, fly your light-ranging planes over Ukraine).
3D from space is a thing, too. Back in 2020, Maxar acquired Vricon for $140M (at a valuation of $300M). At the time, Vricon (a joint-venture between Maxar and SAAB) was making $30M in ARR by algorithmically processing and upscaled 2D satellite imagery, and turning that into 3D data. Last year, Maxar acquired another 3D reconstruction company, Wovenware, which focuses on using AI for 3D creation.
Airborne LiDAR is great for its accuracy, while satellite 2D → 3D, AI-derived reconstruction tools are great for cost. The bottleneck for 2D → 3D is that the data quality isn’t as good. According to Array, it’s 100X worse than what the clusters will collect. A company like Vricon has a significant market, in and of itself, and they were only processing someone else’s pixels.
Combining the best of both worlds is the kicker. As Andrew put it, “What we’ve found in talking to customers is that if you can provide the quality of aircraft imagery at the cost and access of a satellite system, something that’s a drop-in replacement for what customers are already using, then that’s incredibly compelling.”
Array is designing its orbital system (and processing algorithms) to match QL0 LiDAR (quality-level 0, the highest-resolution 3D LiDAR data commonly collected), which means the space-collected 3D data can be immediately piped into applications, such as insurance analytics, infrastructure monitoring, construction management, and self-driving vehicles, which all speak the language of point clouds. These industries already exist; Array’s data is just an improvement.
Plus, the US government and our allies are always hungry for better information about the state of the world. As Maxar and Umbra have shown, you can build great businesses on that revenue alone. But Array isn’t a “Why Now?” for the government, either. I think it’s safe to say the US government will exist with or without Array.
No, the most interesting customer segment for Array from this perspective is one that features an unusual combination: ungodly sums of money and uncertainty about its near-term economic viability. I’m talking about Autonomous Vehicles (AV, or self-driving cars).
AVs are so tantalizing because their own ideal state is so obviously valuable. Cars are a roughly $3 trillion market globally. 46,000 people died in car crashes in the US last year alone. Trucking in the US is a nearly $900-billion annual industry. There are a bunch of big numbers I could keep throwing at you, but the point is this: self-driving cars and trucks have the potential to earn trillions of dollars and save tens of thousands of lives annually.
So it’s not a surprise that investors and the companies they’ve backed have spent something like $100 billion to make self-driving a reality. And it’s finally here, sort of. Cruise, the most widely available self-driving service, is now operating in three markets, and testing in 15 cities:
The tweet provides a hint into why Cruise is only in 15 cities: to enter new ones, it needs fresh 3D maps, which it typically builds through manual data collection, or getting human drivers to drive around every road multiple times while LiDAR scanners on the top of the car collect data on their surroundings.
And they don’t just need to do it when they enter the markets. Maps get stale and need to be refreshed very frequently, which means taking the cars out of revenue-generating service and paying human drivers to cruise around the city again and again. With LiDAR-equipped cars costing $1.5 million, this costs something like $20-30 per mile mapped per car. (Unfortunately, cars can’t map while they’re in self-driving mode; they need the sensors and processing power for the task-at-hand.)
Self-driving car companies need LiDAR QL0 3D maps to operate. Array has back-of-the-napkin backed into the following guesstimate for the world’s leading Level 4 self-driving developers: generating and maintaining HD maps, – just in two to three cities – can cost as much as $1-3 million… per week… per company!
There’s long been a theory around self-driving cars that the technology would be ready long before regulation allowed them to drive around our cities. While certain cities might block self-driving, even San Francisco allows it. It turns out, the bigger threat to the spread of self-driving cars is that it just costs too much money to enter new markets and maintain them.
I think you see where this is going…
When Array Labs is up and flying, they should be able to provide AV companies – startups and incumbent automakers alike – LiDAR QL0-quality 3D maps of the entire country for a tiny fraction of the cost. Plus, those maps would refresh weekly and then daily and then, one day, maybe hourly, as Array sends up more clusters. By subscribing to the Array data, AV companies would improve both their top lines – more cars driving taxi trips instead of mapping, in more markets – and their bottom lines.
In a very real way, Array could be the “Why Now?” for the growth of a market that’s already spent $100 billion to get to this point.
It should come as no surprise, then, that along with the US government, AV is the first market that Array is targeting. They’re already talking with most of the leading players in the space, across urban ridehail, autonomous freight, and automated driver-assist features for OEMs
When you have a product that could serve so many different markets, having a clear strategy, including which markets to serve first, is critical. It determines who you hire, what software you build, and even where to position the clusters.
The thing that’s impressed me most about Array is this: that while Andrew is a technical founder who would have been happy never starting a company, he’s been as meticulous about strategy as he is about engineering. “First time founders care about product, second time founders care about distribution” is the line I’ve heard him say most frequently.
That said, a good strategy isn’t a guarantee. It just gives you the best shot.
What might stand in Array’s way?
Risks
I love the risk sections of these essays on super Hard Startups, because the whole point is taking crazy risks that the founder thinks are actually less risky than most people believe. That said, it would be equally crazy to write an essay on a company that’s >2 years away from launching a novel hardware product into space without acknowledging what could go wrong.
There are a few big categories of risk that I see: Technology, Market, Funding, and Space Stuff. I’ll hit each.
Technology
Array is de-risking as much of its technology on the ground as it can. Successfully creating 3D images with its test rig and algorithms was an important step. But a lot of what Array is doing can’t be fully de-risked until they actually put satellites in LEO.
I’m not a rocket scientist (or a radar engineer), but to my untrained eye, formation flying, communicating radar data between dozens of satellites seems both challenging and impossible to perfectly test on earth. Plus, it’s still premature to write AI/ML tools to exploit and analyze the data.
A lot of things need to not go wrong in order for Array to deliver the high-quality data it hopes to provide customers. As Andrew said, “first time founders focus on product, second time founders focus on distribution.” Array needs to do both. If it can get a cluster of dozens of satellites to stay in formation, talk to each other, and send good data down to earth, it still needs to win customers.
Market
One of the tradeoffs deep tech startups make is technical risk for market risk. Ian Rountree at Cantos defines deep tech like this:
Deep tech = Predominantly taking technical risk (rather than market risk)
If you can build it, they will come. The Array team has had close to 100 customer discovery calls across Big Tech, automotive, insurance, real estate, and other sectors that attest to this holding true here.
There is one piece of the market that’s riskier than the rest, though, and it’s one of Array’s first target markets: AVs.
If you’ve followed the self-driving race somewhat closely, you might have been screaming at your computer reading the last section. I only described one of the two main approaches to self-driving: sensor fusion. As the name suggests, sensor fusion takes data from a bunch of sensors – LiDAR, radar, cameras, and GPS – to understand the car’s surroundings and make real-time driving decisions. Companies like Cruise and Waymo are on Team Sensor Fusion.
But there’s another approach: vision-based. The vision-based approach takes inspiration from the way people drive – we just watch our surroundings and make decisions on the fly – by using cameras and computer vision algorithms to interpret the environment and make decisions in real-time.
There are a number of companies working on the vision-based approach, including George Hotz’s Comma AI, Wayve AI, and, most prominently, Tesla. Tesla’s Full Self-Driving is powered by pure vision, and end-to-end ML (video in → controls out, without any human-programmed rules).
The jury is still out on which approach will win. Most of the industry falls into the sensor fusion camp, but it’s always a risky move to bet against Elon Musk.
The risk, for Array, is that vision-based self-driving doesn’t need high-res 3D maps. If vision-based wins, Array’s data becomes a lot less valuable to AV companies.
Funding
In deep tech, there’s a risk that comes from the timing mismatch between potential future revenue and the real costs a company needs to incur today in order to capture it: “The Valley of Death.”
Array will need to to fund itself through its demonstration launch in 2024, orbital pathfinder mission in 2025, and first cluster launch in 2026.
To date, Array has raised $5.5 million, $500k from YC and $5 million in an October 2022 seed round led by Seraphim Space and Agya Ventures. It will likely need fifteen to twenty million more dollars to get to cluster launch.
In any market, but especially the market we’re in now, venture funding is not guaranteed. According to PitchBook data, venture investment overall is on track to decline 52% from a 2021 high of $759 billion to $363 billion in 2023.
Investment in space technology, however, is holding up better. VCs have invested $4.24 billion in space to date in 2023, on pace to edge out 2022 ($5.4 billion) at $5.8 billion. That would represent a less steep 30% drop from 2021’s highs.
Fortunately for Array, investors seem to have an appetite for space-based SAR. The 2023 number includes a $60 million January Series C for Capella Space, valuing the company at $320 million post-money. Umbra raised its $79.5 million Series B in November 2022, valuing the company at $879.5 million post-money. Iceye raised a $136.2 million Series D in February 2022, putting its valuation at $727.1 million post-money.
Getting to launch is critical, and can take tens of millions of dollars. Prior to launching their first satellites, Iceye, Capella, and Umbra had raised $18.8 million, $33.8 million, and $38.3 million, respectively. Each company raised a larger round of funding within six months of their first launch, once the technology had been de-risked.
Presumably, Array will need to raise an additional $10-30 million before launching its full cluster in early 2026, at which point it should have contracts with both government and commercial customers that begin to contribute meaningful revenue.
This is a solvable risk. After spending time with Andrew and Duffy, and writing this piece, I want to invest, and I doubt I’m alone.
Space Stuff
Finally, there’s one unavoidable risk worth mentioning quickly: Hardware is hard. Space is harder. I had a bunch of paragraphs written on this, but I think it’s self-explanatory. Space junk and rocks can knock satellites out of orbit. Geomagnetic magnetic storms and solar flares could disable them. It can be difficult to find an open slot on a SpaceX rocket. These are risks that all space companies face, and Array does too.
Array has built redundancy into its design — it has dozens of satellites in a cluster instead of one — but space is a harsh mistress. The only way to derisk is to go up.
Those are the big four categories of risk as I see it: technology, market, funding, and space. I’m sure I’m missing some. Array is trying to bring both a novel product and a novel business model to market, simultaneously. Plus, with such a large opportunity at stake, competitors won’t make it easy for Array.
None of them is impossible, though. Array’s team has worked with similar technology before. The self-driving market is unlikely to be winning-approach-take-all, at least for many years. Array is very fundable. And space stuff is hard, but companies pull it off. They do it not because it is easy, but because it is hard.
All of these are risks worth taking, because if Array succeeds, it will quite literally change the way we see the world.
Mapping the Path to Mapping the World
Array Labs is sprinting to bring the earth observation market to its ideal state: near-real-time 3D maps of the Earth.
In just over a year post their YC demo day, Andrew and Isaac will have put together a small but stacked team of eight, built a working multistatic test range and used it to construct 3D images, completed the PDR of the satellites it will send to space, and engaged in conversations with customers at the very top of its two first target industries: defense and AV.
In advance of its demonstration launch next year, during which it will fly four satellites to de-risk formation flying and multistatic capabilities, it’s going to more than double the size of the team. That will be its own sort of test of the theory that the cluster gets more powerful with each additional unit you add.
Array has to move fast. It’s rare to get an opportunity like the one it has. Advances in AI and ML have increased the demand for 3D point clouds just as launch costs are tumbling, cubesats are getting cheaper and more capable, and RF technology has advanced enough to make multistatic SAR possible. The conditions were ripe for Andrew’s crazy idea, and the company needs to capitalize before others catch on.
If Array can execute, it has the potential to tap into billions of dollars of existing spend and enable new markets that weren’t possible before. If it plays its cards just right, it will be able to disrupt the traditional earth observation market, introduce a new higher-margin business model, and use that model to protect its margins against inevitable competition with scale economies and network effects or switching costs. Array can and should move fast because its strategy is so clear.
Of course, there will be challenges. Startups are hard. Hiring excellent talent is hard. Getting teams to move fast together is hard. Hardware is hard. Space is hard. Formation flying and inter-satellite communication is hard. Selling to DoD is hard. Establishing new markets is hard. EO has proven to be a very hard market to make money in. Everything Array is doing is hard.
But holy grails are, by definition, hard. All that difficulty is a sign that Array is on the right track. And seemingly impossible things are happening more and more frequently. I’d put my money on Array to pull this off.
And if it does, the implications are enormous. Array has the potential to build a multi-billion dollar business that enables multiples more value than that to be created. That’s the real goal.
In that conversation Andrew and I had about moats back in July, he said that one of the biggest questions he was working on was, “Is there a way to deploy this to as large a group as possible without capturing extraordinary profits?”
Building the 3D map of Earth is the holy grail not because of the map itself, but because of what people can do with it. It’s more useful to humanity the more people can play with it and build on it. Customers in existing markets – like AV companies, the DoD, and Big Tech – should be able to get better performance for less money, but Andrew is thinking about how to give the data to universities, non-profits, and entrepreneurs as cheaply as possible.
“I want to generate value first and foremost,” he told me, “and I’m OK not capturing 99.99% of that.”
That could be worrying for investors until you realize how much value near-real-time 3D maps of the world might generate. AV is a multi-trillion dollar industry in waiting. So is autonomous trucking. Solving climate and deterring conflict are existentially important. And opportunities in energy, resource management, AR, urban planning, agriculture, construction, and infrastructure, logistics, and disaster response add up to trillions more dollars, and an incalculable impact on humanity. The more widely the maps are used, the better. There’s plenty of value to go around.
I already ripped the band-aid with a Nvidia comparison earlier, so I’ll go with it: Nvidia has built a trillion dollar company by making AI possible. If AI is as valuable as even its more measured proponents think it can be, that trillion represents a small fraction of the overall value to humanity. I think a similar dynamic might be at play here, although only time will tell what order of magnitude we’re dealing with.
But I’m getting ahead of myself. Array still needs to launch its first cluster, sign its first customers, and build a business. The idea works on paper. On paper, it’s one of the most compelling startups I’ve found. But there’s only one way to know if it works in practice…
But to build the 3D map of the world, you need to send dozens and dozens of satellites to space. It’s a crazy idea, but I’m betting it’s just crazy enough to work. It’s going to be fun watching Array pull it off over the next couple years.
Here’s to the crazy ones. The ones who redraw the boundaries.
Thanks to Dan for editing, and to Andrew and Duffy for teaching me the ins and outs of SAR and letting me tell the Array Labs story!
That’s all for today. We’ll be back in your inbox with a Weekly Dose on Friday. Go get it this week.
Thanks for reading,
Packy
math error, I think
" At around $500 per square kilometer (apologies for the mixed units, but we’re going with industry standards), a single US collection would be $5 billion." That's about right, in that the US is about 10M km^2. But then, "current retail pricing of around $50/sq. km, it would only cost you $150 million to cover the US, or $7.7 billion to cover the whole Earth. ". But if it'S 10x cheaper, it should cost $500M to cover the US. You seem to have the right multiple of US/earth surface area (earth:US :: 51:1), so the cost for the earth should be ~$25B.
Fascinating article. Only thought is why does the Array multi static SAR ring need to be dedicated ? Ie if know position of satellites in LEO then why not add capability,to say, thousands of starlink sats. I am guessing the ring shape and close Comms between sats in ring critical.