Welcome to the 1,435 newly Not Boring people who have joined us since last Monday! Join 80,283 smart, curious folks by subscribing here:
🎧 To get this essay straight in your ears: listen on Spotify or Apple Podcasts
Today’s Not Boring is brought to you by... Sprig
Sprig is your all-in-one product research platform. Many of the world’s leading tech companies including Square, Adobe, Loom, Retool, Opendoor, and Chipper use Sprig to capture insights from their customers and build better products.
Recently, Sprig rolled out two new solutions: video interviews and concept testing. Now, companies can have async video conversations with users, and can even share Figma designs at the same time to get rich feedback on concepts and prototypes that are still in development. If you build products, you should just try it for yourself. It’s all of your product research tools, in one place.
The Sprig team knows that once teams start using it, they don’t stop, so it’s offering a free onboarding and 30-day trial to any enterprise company (~100,000+ users).
Hi friends 👋,
Happy Thursday! When I invested in ScienceIO early in Not Boring Capital’s life, I said that I’d write an investment memo on the company when they announced the raise. That was purely selfish: I figured that writing an investment memo would force me to figure out wtf the company actually does. Plus, I get to tell you about a company that I’m confident will be a unicorn and will help extend and save millions of lives, with *science*.
Let’s get to it.
The Model of Everything
Investment Memo on ScienceIO
In February 2018, Swiss pharmaceutical giant Roche bought NYC-based startup Flatiron Health for $1.9 billion. Flatiron, founded in 2012 by Nat Turner and Zach Weinberg, collects and organizes real-world clinical oncology data from the thousands of community clinics, medical centers, and hospitals across the country where it was previously siloed, and delivers clean data to drug development companies and researchers working to fight cancer. That’s an amazing mission and the sale was a big outcome, but Flatiron’s model had a challenge: people.
Flatiron needed to hire tons of experts (oncologists and oncology nurses) to extract data from electronic health records into databases, where it could be used, understood, and manipulated to drive outcomes. Luckily, the Flatiron team was willing to take on that work (and cost) to help transform the way cancer is understood and treated, but the difficult unit economics hold other entrepreneurs back from creating the next “Flatiron for x.”
Will Manidis, the co-founder and CEO of ScienceIO, told me that his big vision is to change that. “We want to make it 100x easier to start a company that looks like Flatiron. There should be people building Flatiron for everything.”
So how do you do it? It’s as simple and as impossibly difficult as making healthcare data computable.
That means transforming the 2 zettabytes of healthcare data created each year, most of which is messy and unstructured, into structured data that can be searched, analyzed, and mined for insights. That’s what ScienceIO does.
ScienceIO decodes the language of medicine to unlock the full potential of healthcare data. Its industry-leading clinical Natural Language Processing (NLP) platform structures data in real-time to enable search, analysis, and insight generation.
Yesterday, ScienceIO announced that it raised an $8M seed round from investors including Section 32, Sea Lane Ventures, Quiet Capital, Lachy Groom, Josh Buckley, Scott Belsky, Todd Goldberg, Rahul Vohra, Turner Novak’s Banana Capital, Ankur Nagpal, Austin Rief, Jack Altman, Pipe founder Harry Hurst, Earl Grey Capital, Swell Partners, The Dorm Room Fund (where Manidis was a Managing Partner), and Not Boring Capital. It’s using the money to make healthcare data computable.
I’ll get this confession out of the way upfront, and it may shock some of you: I do not personally know how to make healthcare data computable at scale. But Will and co-founder Gaurav Kaushik do, and today, I’m going to do my best to explain why I think they have the potential to build one of the most valuable companies in the Not Boring Capital portfolio.
Let’s bring this company out of stealth by covering:
The Investment Thesis
The Challenge: Unstructured Zettabytes
The Team: Building on a Strong Foundation
The Products: The Model of Everything
The Business: 💰📈
The Future: Patient 360 and Healthcare Infrastructure
The Investment Thesis
First things first: why did I invest in ScienceIO?
In two years, ScienceIO has already built the largest healthcare AI training data set and is building infrastructure that companies and researchers across the industry can use to save time and money, develop new pharmaceuticals, treat patients better, and drive improved outcomes.
The opportunity is massive. There is $4 trillion in annual healthcare spend in the US alone -- 17% of GDP -- and the system still relies on messy, unstructured data for everything from drug development to patient care. Since data underpins everything in healthcare, ScienceIO can sell to nearly any company in healthcare: it’s already working with large insurers, clinical trial research providers, pharmaceutical companies, and an academic medical center, highlighting the breadth of potential deep-pocketed clients.
The quality of clients they’re working with already speaks to the fact that they really do have the best product in the market. Now that it has built the data set and models, ScienceIO is able to sell high-ACV, high-margin contracts and scale revenue very rapidly.
More importantly, better data will mean better health for millions of people.
So why hasn’t this been done before? All of this is really hard. No one’s ever come close to what ScienceIO has pulled off.
The Challenge: Unstructured Zettabytes
Think back to the last time you went to the doctor’s office and had to get a prescription. Your very smart doctor, who spent years and years and hundreds of thousands of dollars on medical school and just figured out what ailed you and how to fix it in five minutes, tells you they’re going to prescribe you something, whips out a pad of paper, and scribbles this:
Healthcare data is a uniquely tricky beast. While not every document looks like a prescription note, more than 80% of healthcare data is unstructured, living in a digital format that requires manual labor to analyze, in images, or, like that script, in handwritten notes. Will put it simply: “Healthcare runs on notes, scans, and PDFs.”
And there is a TON of it. Each year, the healthcare system creates two zettabytes of data. If you don’t know what that even means, don’t worry, I didn’t either. But I looked it up. A zettabyte is a trillion gigabytes. All of humanity only started moving a zettabyte of data across the internet -- with all of the shows, songs, tweets, likes, and more -- somewhere between 2012 and 2016, depending on when you believe we entered the Zettabyte Era.
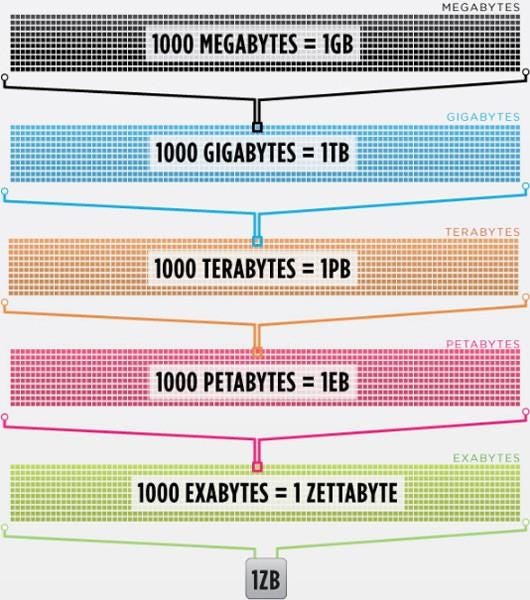
So healthcare today produces twice as much data each year as all of humanity produced in a year less than a decade ago. That data comes from a ton of different sources:
Wearables, medical devices, and sensors. This data, from all the things we and our doctors put on our body to track our health, is the relatively clean and easy stuff. It’s digitally native.
Electronic Medical Records (EMR) / Electronic Health Records (EHR). As we covered when I wrote about NexHealth, this data is siloed, difficult to access, and messy.
Clinical Data. Every time you go to the doctor’s office, a specialist, the hospital, a telehealth visit, or receive medical care in any way, data is created, be it scribbles on paper or a more thorough writeup.
Insurance Providers. Millions of medical insurance claims are filed each year, each of which produces data on what was paid for and often why.
Medical Imaging. MRIs, CT Scans, X-Rays, and other diagnostic imaging techniques create images that need to be stored, understood, and structured.
Clinical Trials. ClinicalTrial.gov tracks 392k registered clinical studies worldwide, up from just 2,119 in 2000, 51k of which have posted results. Each trial has data from dozens, hundreds, or thousands of people, each with their own unique attributes.
Genome Registries. The results from 23andMe, or any other genomic testing, produce a ton of valuable data that can inform healthcare decisions on the individual and population level.
That’s most of it, but there’s more. The system is woefully unprepared for all of that data. Historically, the healthcare industry has invested in protocols for the structured data it has, digitizing analog data but leaving it unstructured, and brute-forcing the unstructured data problem through manual labor. These solutions have plateaued.
This is the challenge that Flatiron faced, and that was just cancer, in just the US, with just 280 community cancer centers, seven academic cancer centers, and 20 pharmaceutical companies. Flatiron employs 2,500 people, many of whom manually transcribe and tag data. It’s impossible to do that for all of the healthcare data in the world, particularly when it’s growing exponentially.
In order to make progress in healthcare, we need to turn all of that messy, unstructured data into clean, structured data that researchers, pharmaceutical companies, doctors, insurance companies, and other medical professionals can actually use.
That’s where Will and Gaurav come in.
The Team: Building on a Strong Foundation
If anyone was going to build a venture-backed startup that uses NLP to make sense of healthcare data, it was going to be Will Manidis.
ScienceIO is the marriage of machine learning and healthcare. Will is the marriage of machine learning and healthcare. It was meant to be.
When Will was in high school, a time when most of us were just trying to get good grades and get into college, he was using large scale NLP to study cross-language allusions in early classical texts. NLP, or Natural Language Processing, sits at the intersection of machine learning and linguistics. Using NLP, computers can understand human language as it’s written or spoken. Siri and Alexa, for example, both use a combination of speech recognition and NLP to figure out what you’re saying and then make sense of it.
Young Will ran early classical texts, like the Virgil’s Aenied or Silius’ Carthaginian Debates through NLP models to pick up cross-language allusions that humans couldn’t. Kid was wicked smart.
As wicked smart Wills do, Will studied at a college right outside of Boston.
He attended Olin College, an engineering-focused school founded in 1997. While in school, he worked two jobs: one, as a Partner and then Managing Partner at student-run venture fund Dorm Room Fund, and the other at therapeutics company Immuneering. Will told me he jumped into healthcare thanks to his experience spending a lot of his youth sick. At Immuneering, while, remember, still in college, he built out systems to accelerate the speed of clinical literature. After a year at Immuneering, he joined Cambridge-based Foundation Medicine, a decision insights company that helps doctors connect their patients to the best cancer treatment options, as a Visiting Researcher.
At Foundation, Gaurav Kaushik was leading the real world data (RWD) team, which he started, focused on leveraging patient data to create solutions that advance patient care. Gaurav, too, has an absurdly strong background that laid the groundwork for what ScienceIO would become. I’m going to bullet out his LinkedIn so that when we talk about the crazy shit that ScienceIO is pulling off, you actually believe it:
B.S. in Biomedical Engineering at Columbia, where he was an undergraduate researcher
Ph.D. in Bioengineering and Biomedical Engineering from UC San Diego, where he was a graduate student researcher at the Stanford Consortium for Regenerative Medicine (check out his dissertation on Cytoskeletal Regulation of Form and Function in Aging Myocardium)
Health Data Science Fellow at Insight Data Science, a fellowship for PhDs who want to pursue a data science career
Postdoctoral Research Fellow at Harvard Medical School, where he was an NIH Organ Design and Engineering Fellow “working on next generation organ-on-chip solutions”
Scientific Program Manager and Director, Advanced Concepts at Seven Bridges, a startup that creates cloud-based platforms for analyzing petabytes of biomedical data.
I feel sufficiently underachieving at this point; luckily, that takes us back to Foundation, where Gaurav was running the RWD team. In 2018, Will joined Gaurav’s team to help develop AI tools that could identify patients with unmet needs and predict outcomes. (That same year, Roche acquired Foundation for $2.4 billion… sensing a theme). To do so, they built comprehensive views of patient health and outcomes and were able to understand what moves the needle for patients like never before.
As one example, their team looked at patients with triple negative breast cancer (TNBC), a very harsh form of breast cancer that particularly affects women of color. They manually aggregated data for those patients and did an analysis that showed that they responded very well to a particular class of cancer therapies, but those therapies were off-label and very expensive. When they presented the data, both internally and at conferences, people were surprised. There were no active clinical trials for the therapies they’d identified in TNBC. Without the team and resources they had at Foundation, they never would have known that there was a therapy that could potentially work for these patients that should be accelerated for clinical studies and FDA approval. They were saving lives with data.
After that experience, Will and Gaurav started asking themselves, “Who else are we missing out there? What stories aren’t being told because the data isn’t organized? What if we could tell these stories at scale?” They decided to find out.
In 2019, Will dropped out of Olin to become a Thiel Fellow. (Fun fact: since he was already gainfully employed and didn’t need the money to survive, he took the $100k and invested $10k in 10 companies. He’s built an incredibly strong portfolio.)


They both left Foundation, and launched Cascade.Bio (which they later rebranded to Science.io in a story for another day).
The plan: build the “Model of Everything” for healthcare.
The Product: The Model of Everything
What does the Model of Everything look like for healthcare?
In science fiction, the Model of Everything Healthcare AI might scan a patient’s body, run full diagnostics, sequence her DNA, analyze the latest clinical trials, and deliver a personalized combination of medications and nanobots to cure anything that ails her. And maybe ScienceIO will be a step in that direction.
But the Model of Everything we’re talking here about is this: A single model that can take any healthcare artifact -- a physician’s note, a diagnostics report, a clinical trial protocol, a research paper, etc... -- and deliver structured data, in real-time.
When a typical company runs machine learning models -- say Uber’s pricing algorithm -- it’s pulling from its own database, which is structured the way that the company’s data scientists and engineers designed it.
Uber takes inputs from its own apps, like the number of people requesting a ride in a certain area and the number of available drivers in that area, throws them in its database, and runs models on top that set the price that optimizes for whatever Uber is optimizing for (ie. more revenue or lower wait times). The data science is hard, but they’re starting from structured data.
The healthcare system is a much more complex beast. We covered a lot of that complexity when I wrote about Antara Health, Cityblock Health and NexHealth. Simply, though, healthcare is focused on making people healthier by delivering the right treatment at the right time in a cost-effective way.
Leaving aside the complexity in the system itself for a minute -- red tape, insurance, HIPAA -- the actual treatment part is challenging because everyone is unique. One-size-fits-all doesn’t work in medicine like it would in, say, ride hailing, where supply is fungible. Just get me a car. To deliver the right treatment at the right time in a cost-effective way requires understanding the entire patient population’s medical histories, genetics, social determinants of health, diagnoses, treatment plans, and more.
There are 7.9 billion people on the earth and millions of medical conditions, devices, drugs, therapies, biomarkers, and genes. All of that data comes from disparate sources and in different formats. 80% of it is unstructured. Plus, healthcare has its own language and syntax. People go to medical school for decades to learn it all.
So the job of the Model of Everything is to be able to take any piece of messy, unstructured healthcare data that people feed into it, and turn it into structured data that healthcare professionals can use to deliver the right treatment, at the right time, in a cost-effective way.
ScienceIO is pulling it off in three steps: Data Labeling, Model, and Annotation.
It all starts with data labeling. In Scale: Rational in the Fullness of Time, I explained:
ML models take data as an input and let the machine learn its way into figuring out the right code. Without data, there is no ML or AI, and bad or mislabeled data is worse than no data at all. “Garbage in, garbage out.”
To build the Model of Everything in healthcare, you need a massive set of labeled data, but Will told me that when they “surveyed datasets for training AI to read healthcare information, most datasets were focused on narrow use cases (e.g. just drugs, or generic diseases) and most ranged from 500-5000 labels (the largest had fewer than 100k).”
That was nowhere near big enough.
Plus, all of the labels in the datasets they discovered were far too surface level. They could tell you that a piece of text referred to a chemical, but not which chemical. Practically useless. In order to fully structure healthcare data, they needed to understand patient data with precision, which means connecting the actual drug, dosage, and other context, not just recognizing the topic.
After months of searching, Will and Gaurav realized that there was no reliable data structure for clinical NLP. They decided to build it themselves.
Building a dataset as large as they required with human labelers would be incredibly expensive and incredibly slow. In order to capture the millions of variables that inform patient care with enough specificity to be useful, they’d need hundreds of millions of labels to achieve greater than 99% accuracy. For a team of 100 data labelers, it would take several years and up to a billion dollars to generate enough data to scratch the surface of healthcare information.
That solution wouldn’t work. They needed more than training data. They needed a system that would take any healthcare data -- from customers, new research, existing datasets -- and make it learnable.
So they built Tycho.
Tycho
It worked. Tycho is the world’s only platform that can automatically turn unstructured healthcare data into richly-labeled data at greater than human accuracy. Tycho is capable of labeling specific medical conditions, devices, drugs, surgeries, treatments, biomarkers, genes, and more.
With Tycho, ScienceIO built the world’s largest and highest quality dataset for training healthcare AI. Today, the dataset has over 2.2 billion labels in over 20M documents spanning clinical trial records, physicians notes, and clinical research papers.
That’s 22,000x larger than the previous largest healthcare dataset, it scales rapidly, and it’s still growing. Tycho grew from 40M labels in early 2020 to 2.2 billion labels and over 20+ clinical ontologies integrated in 2021. (Ontology is a fancy word for a set of medical terminologies and the relationships between them. Drop it casually in conversation today.) In the past 12 months, its data has grown 50x.
Plus, Tycho maps out advanced logic in the text. Remember, healthcare has its own language and syntax, so Tycho needs to understand that logic and adjust labels accordingly.
Advanced logic lets ScienceIO’s customers identify specific lines of therapy, dosages, biomarker levels, performance metrics, and more, the information they need for actionable analysis.
Kepler
In the 16th Century, a wealthy Danish astronomer named Tycho Brahe hired a 27-year-old German astronomer named Johannes Kepler as his assistant, and asked him to define the orbit of Mars. While Tycho was alive, Kepler was unsuccessful. Tycho withheld the lifetime of astronomical observations he’d made from his assistant because they went against his Earth-centric view of the universe. But upon the elder astronomer’s death, his papers were passed down to Kepler. Kepler used the data that Tycho had collected to correctly define the orbits of the planets around the sun and discover the three laws of planetary motion.
Fast-forward 500+ years, and Tycho and Kepler are at it again. Tycho collects the data, and Kepler learns from it. In this case, Kepler is ScienceIO’s platform for parallelized, multi-GPU training of massive language models. Together, Tycho and Kepler take tons of unusable data and turn it into models that perform clinical-grade information extraction in real-time.
They believe that large language models, like Kepler, are the best way to transform healthcare data at scale, for a few reasons:
Language models turn text into code.
NLP is developing rapidly, growing the number of tools and techniques available.
Language models get better with more data -- as they go from 2.2 billion labels to 100 billion labels, the algorithms keep getting better.
Language models transfer between data sets, meaning they can learn from big, public datasets and fine-tune down for specific use cases where less data is available.
The end result is that, with Tycho and Kepler, ScienceIO built a single model that can ingest any healthcare artifact -- physician notes to research papers to diagnostic reports -- and spit out clean, structured data in real-time.
ScienceIO’s customers can access all of this magic via an API so that they can build the model of everything right into their workflows, or through custom solutions.
For the third and final step, ScienceIO brings humans back into the loop. Annotate, its AI-assisted data-labeling and training platform, lets users upload their own notes, PDFs, and Office docs, which ScienceIO’s models pre-label. Users can validate, change, add, or remove labels, which captures each user’s intuition over time.
For example, a doctor might bring up her notes, label patient data with AI assistance, and automatically train algorithms that help predict which treatments will work, based on both the data and her own experience. Her expertise wouldn’t only help her patients, but would feed back into the model to help all similar patients (maybe we even get a $DOCDATA coin that rewards the doctor every time her input is used elsewhere?!).
ScienceIO is building towards a world in which human-AI teams turn medical expertise into code that can be systematized across the industry.
It’s still early. For now, ScienceIO is making its customers faster, smarter, and more efficient. Annotate has an intervention rate of <2%, meaning that most AI-generated labels are approved with fewer than 2% needing to be adjusted. Plus, they’ve observed a 20x speed up with AI-assisted labeling versus manual labeling. This is a similar idea to the efficiencies that Scale gets by using AI to pre-label and humans to confirm and adjust.
Taken together, Tycho, Kepler, and Annotate form the Model of Everything in healthcare with room for human expertise. The good thing about Models of Everything is that they have a lot of potential customers.
The Business: 💰📈
Phew, I survived the science and AI 😅 Still with me? Now we’re back on familiar footing: business model.
ScienceIO is a venture business’ venture business: high upfront costs, improbable to pull off, but if you do, low marginal costs and high margins. Over the past two years, ScienceIO pulled off the improbable and de-risked the technical side of the business, building a Model of Everything for healthcare that no one has ever been able to build. Now, it’s time to sell.
For such a technically sophisticated product, ScienceIO’s business model is relatively simple:
ScienceIO invested two years and millions of dollars into building the Model of Everything.
It sells its model to clients via enterprise deals, and is about to launch a self-serve API for developers.
Enterprise deals are longer sales cycle and require some integration work, so they begin with a 3-month pilot in the six figures of revenue and scale to multi-million dollar contracts. Margins on these deals are very high.
Self-serve API deals will be nearly pure margin. ScienceIO charges for API deals on a pay-per-usage model; i.e. every page that a user runs through the model, they pay a small amount of money.
Through Annotate, users will improve ScienceIO’s algorithms as they pay to use the product, giving it increasing returns to scale.
Because of the breadth of ScienceIO’s model, it’s able to serve a wide swath of customers. Its pilot customers include a top insurance company, the largest clinical trial infrastructure provider, a discovery-stage pharmaceutical company that’s repurposing cancer therapies in an emerging therapeutic domain, and an academic medical center seeking to identify and understand rare cancer patient populations from EHR and genomic data.
It’s telling that ScienceIO is signing cancer-focused customers despite the fact that they’re not specifically focused on cancer while others are. The non-medical-non-data-scientist leap my brain is making is that given how interconnected everything that impacts our health is, a more general model will actually outperform more specific models, even in those specific models’ focus area.
If that’s the case, ScienceIO’s total addressable market is unimaginably large. Selling the model that best helps make sense of huge amounts of data for all verticals within a $4 trillion industry in which data creation is doubling every year is a great place to be. And once some customer start using and seeing results from ScienceIO, others will have no choice.
Take that top insurance company, for example. They’re using ScienceIO to digitally transform their manual claims adjustment workflow. Instead of adjusters reading 100+ page PDFs, ScienceIO is able to digitize, analyze, and summarize each claim and layer on intelligence that flags severe cases and potential fraud. When that company grows profits by decreasing labor costs and slashing fraudulent claims, its competitors will have to follow suit. They won’t be able to build this themselves. They’ll have to work with ScienceIO.
Whether ScienceIO is selling enterprise deals with integrations or self-serve API deals, it’s product sits in the API-first sweet spot:
If anything is more in the “needs to get done but we don’t want to waste our time doing it” bucket, it’s reading insurance claims (or using highly-skilled, high-priced oncologists to label data). Those are things you want to pay someone else to do, faster, cheaper, and more effectively.
With strong early traction, ScienceIO is pouring on the gas. Each one of its investor updates is better than the last, with product and sales updates pacing ahead of schedule. Its enterprise pipeline is growing 75% MoM (again, each of those deals represents hundreds of thousands or millions of dollars in high-margin revenue) and its self-serve API waitlist is growing fast into launch.
And they’re really just starting to build out the sales motion: yesterday, the company announced that it brought on Chuck Smolky as its first Chief Commercial Officer. Smolky was previously a Vice President at Optum and Aetna, and a SVP at Health Fidelity. That kind of hire, this early, shows the maturity of the team and Will’s ability to see around corners. The last time we spoke, he told me that he was happy with the company’s growth but already preparing for the point at which high-growth companies level off a couple of years from now.
Building a top-notch commercial team is one way to stave it off. Another is to keep building more irreplaceable products.
The Future: Patient 360 and Healthcare Infrastructure
Let’s recap. In two years, ScienceIO went from an idea to:
The world’s largest healthcare dataset (by far)
A platform for automated labeling of healthcare data at scale with expert intervention
AI that can take any sort of healthcare artifact and spit out structured data using 9 million healthcare concepts
A soon-to-launch API with which developers can access all of the above in a few lines of code
An impressively mature and fast-growing list of customers across healthcare verticals
A product that improves with usage and widens ScienceIO’s lead
Obviously, Will and Gaurav had a head start. Gaurav’s academic and professional history seems to have been designed for just this purpose. Will was running NLP on healthcare data in the womb. But still, it’s really fucking impressive.
So what’s next? Beyond giving customers tools to clean and use their data, ScienceIO wants to build rich patient profiles from all of the disparate data sources it uses. They call it “Patient 360,” a single source of truth for a patient’s health.
Imagine if every time you went to a doctor’s office, got your blood taken, went to the ER, participated in a study, got your DNA sequenced, wore a fitness tracker… it all showed up in one place, and kept updating every time something changed. People could keep their own records, like a personal healthcare passport, and developers would be able to build on top of Patient 360 to build applications that improve patient outcomes. That would be a huge leap.
I have no doubt that ScienceIO is going to be a massive business. If this isn’t a unicorn in the next couple of years I will manually label insurance claim data for a week.
But the more exciting piece of all of this is that they’re creating tools that businesses and entrepreneurs can use to actually make people healthier, clean up the healthcare system, and save lives.
After writing about NexHealth and now ScienceIO, I’m convinced that healthcare will see the kind of change in the next decade that fintech has in the last one. We’ll get dramatically improved patient experiences, more personalized treatment, and increased healthspans. ScienceIO will be a crucial piece of the infrastructure on top of which that happens. It will let the next 100 Flatiron Healths bloom, and reshape the way that humans understand and treat devastating and debilitating diseases.
Here’s to Science.
How did you like this week’s Not Boring? Your feedback helps me make this great.
Loved | Great | Good | Meh | Bad
Thanks for reading and see you on Monday!
Packy
Great Insight! But, I believe elephant in the room was not addressed. I wonder, how folks at ScienceIO are accessing the unstructured data? Xrays/Scans/hand prescriptions these are private info. Where do they get their inputs from?
Thats great