Welcome to the 1,160 newly Not Boring people who have joined us since Monday! Join 58,051 smart, curious folks by subscribing here:
🎧 To get this essay straight in your ears: listen on Spotify or Apple Podcasts (in a little bit)
Today’s Not Boring is brought to you by… Fundrise
Listen, the stock market can be great. Sometimes everything’s green and you look like a genius.
But the stock market can also be unpredictable. YOLO’ing all of your money isn’t a strategy. You should give yourself some peace of mind by diversifying your portfolio.
That’s where Fundrise comes in. They make it easy to invest in private market real estate, an alternative to the stock market, but one that used to only be accessible to the ultra-wealthy. Fundrise changed that. Now, you can access real estate’s historically strong, consistent, and reliable returns with just a $500 minimum investment.
Diversification is a beautiful thing. Start diversifying with Fundrise today.
Hi friends 👋 ,
Happy Monday! Hot Vax Summer is in full-swing, and while all of you are out trying to remember how to party, I’m sitting in a basement doing my darndest to expose myself as a fraud.
My job is to go out to the edges and try to explain things that seem crazy and complex, first to myself and to all of you in the process. I’m not writing as an expert, but as someone who’s taking you along on my own exploration. I’ll get some things wrong, and you’ll call me out, and hopefully we all get a little smarter. In the process, two overarching themes for Not Boring have emerged:
Everything is going to be crazier than we can imagine. Things seem wild right now, but if history is any indication, this period is going to look quaint in a decade and antique in a century. We need to be prepared.
There are still business and strategy principles that underpin even the craziest seeming ideas and businesses. Web3 is really about network effects. Zero-knowledge proofs are about long-standing consumer preferences for convenience and privacy. Even the most futuristic-seeming tech can become commoditized in a handful of years; the businesses built on them still need moats.
Today’s essay is about Scale AI, a company that started with a non-obvious wedge into a large market that’s growing and evolving rapidly, and that will one day grow and evolve faster than humans can comprehend. It’s a company you should know about:
At just five years old, Scale was recently valued at $7.3 billion.
It’s (actually) like Stripe: leveraging a seeming commodity -- data labeling -- as a wedge into a key position in the rapidly growing and evolving AI and ML space.
By building infrastructure, Scale has a chance to help grow AI/ML usage from only 8% of companies today, to all companies within the next two decades.
I’m certainly not an AI/ML expert. I will certainly get some things wrong and welcome feedback. I’m just taking you along on my learning and diligence.
A note: Not Boring occupies a unique and tricky position. I write about the ideas and companies that fascinate me most and best fit the themes above, but I don’t want to sit passively and watch, so I often invest in the companies I see as best-positioned to bring about and capture value from the trends, too. If I were a journalist, this would be frowned upon. But I’m not. I’m just trying to dive in as deeply as I can to understand all of this better, bring back what I learn, expose my thought process (and potential conflicts) openly, and help shape the future in a small way.
Full disclosure: I’m an investor in Scale, but didn’t receive compensation from Scale for this piece.
Let’s get to it.
Scale: Rational in the Fullness of Time
^^ Click to jump straight to the web version ^^
On Thanksgiving last year, Alexandr Wang posted his first essay to Substack. The essay, Hire People Who Give a Shit, was good. So was the next one, Information Compression, which he wrote on December 5th. Both provided well-reasoned glimpses into the way Wang runs his company day-to-day. But the essays weren’t the most illuminating thing about the Substack. The name he chose for it was:
Rational in the Fullness of Time
The name is fitting given Wang’s long-term focus at his company, Scale. Scale has spent its first half-decade focused on the unassuming first step of the machine learning (ML) development lifecycle -- data labeling or annotation -- with a belief that data is the fundamental building block in ML and artificial intelligence (AI). For a while, that has meant that the company has looked like a commodity services business.
Scale exists to “accelerate the development of AI applications” by “building the most data-centric infrastructure platform.” Its core belief, and the assumption on top of which the business is built, is that “Data is the New Code.”
Scale wants to be to AI what AWS is to the cloud, Stripe is to payments, Twilio is to communications, or Snowflake is to Data Analytics.
Now, of course Scale wants to be like those companies. Who doesn’t? But it’s on a credible path:
Scale spent its first four years focused on annotating data for use in AI/ML models. Now, it’s expanding downstream to develop the models itself and eat other pieces of the AI/ML value chain.
It surpassed $100 million Annual Recurring Revenue (ARR) in 2020, just its fourth year in operations, and continues to more than double year-over-year.
Its clients range from the US Department of Defense to PayPal to all of the major Autonomous Vehicle (AV) companies and largest tech companies.
Just five years old, it’s valued at $7.3 billion after a recent $325 million funding round co-led by a triumvirate of top growth investors Greenoaks Capital, Dragoneer, and Tiger Global.
It brought on former Amazon exec Jeff Wilke as a special advisor to the CEO.
Scale builds infrastructure, which looks pretty unsexy, until it doesn’t.
In a decade, if Scale is successful, any company that wants to build something using AI or ML will just stitch together five different Scale services like they stitch together AWS services to build something online today. Scale could collect or generate data for you, label it, train the machine learning model, test it, tell you when there’s a problem, continue to feed it fresh, label data, and on and on. Via Scale APIs, companies of any size will be able to build AI-powered products by writing a few lines of code.
Take a second to appreciate that: within a decade, AI, long the stuff of sci-fi writers’ imaginations, will be as easy to implement as accepting a credit card is today. That’s mind-blowing.
But that’s in the future. First, data.
When Wang and co-founder Lucy Guo founded Scale out of Y Combinator in 2016, the company was called Scale API and its value prop was essentially that it was a more reliable Mechanical Turk with an API. They started with the least sexy-sounding piece of an incredibly sexy-sounding industry: human-powered data labeling.
Customers sent Scale data, and Scale worked with teams of contractors around the world to label it. Customers send Scale pictures, videos, and Lidar point clouds, and Scale’s software-human teams would send back files saying “that’s a tree, that’s a person, that’s a stop light, that’s a pothole.”
By using ML to identify the easy stuff first and routing more difficult requests to the right contractors, Scale could provide more accurate data more cheaply than competitors. Useful, certainly, but it’s hard to see how a business like that … scales. (I’m sorry, but I also can’t promise that will be the last scale pun).
Scale’s ambitions are obfuscated by its starting point: using humans to build a seemingly commodity product. A bet on Scale is a bet that data labeling is the right starting point to deliver the entire suite of AI infrastructure products.
If Wang is right, if data is the new code, the biggest bottleneck for AI/ML development, and the right insertion point into the ML lifecycle, then the brilliance of the strategy will unfold, slowly at first then quickly, over the coming years. It will all look rational in the fullness of time.
Scale has a high ceiling. It has the potential to be one of the largest technology companies of this generation, and to usher in an era of technology development so rapid that it’s hard to comprehend from our current vantage point. But it hasn’t been all clear skies to date, and the future won’t be easy either. It will face competition from the richest companies and smartest people in the world. It still has a lot to prove.
In either case, Scale is a company you need to know. It’s also an excellent excuse to dive into the AI and ML landscape and separate fact from science fiction. It’s looking increasingly likely that AI will find itself in the technology impact pantheon alongside the computer, the internet, and potentially web3.
The combination of all of those technologies will change the world in unpredictable ways, but one thing’s certain: the world only gets crazier. We’re at an inflection point, so let’s get ready by studying:
The State of AI and ML
Getting to Scale.
Scaling Like Stripe.
The Bear Case for Scale.
The Bull Case for Scale.
Scale’s Compounding Vision.
Before we get to Scale, though, we need to get on the same page with what AI and ML are.
The State of AI and ML
I’ll start with the punchline, and then get to the joke: whether you call it AI or ML, it’s useless without good data.
Now the joke. There’s a set of jokes among technical people whose premise is that machine learning is just a fancy way of saying linear regression and that artificial intelligence is just a fancy way of saying machine learning. No one made the joke better than this guy:

The idea is that machine learning is the real thing, written in the Python programming language, and AI is just hype-y way of saying ML that people use to fundraise.
There are differences, though. I asked my friend Ben Rollert, the CEO of Composer and my smartest data scientist friend, how he would define the differences between AI and ML. His response seems pretty representative of the general conversation:
AI is a broad bucket of “algorithms that mimic the intelligence of humans,” some of which exist today in machine learning and deep learning, and some of which still live only in the realm of sci-fi.
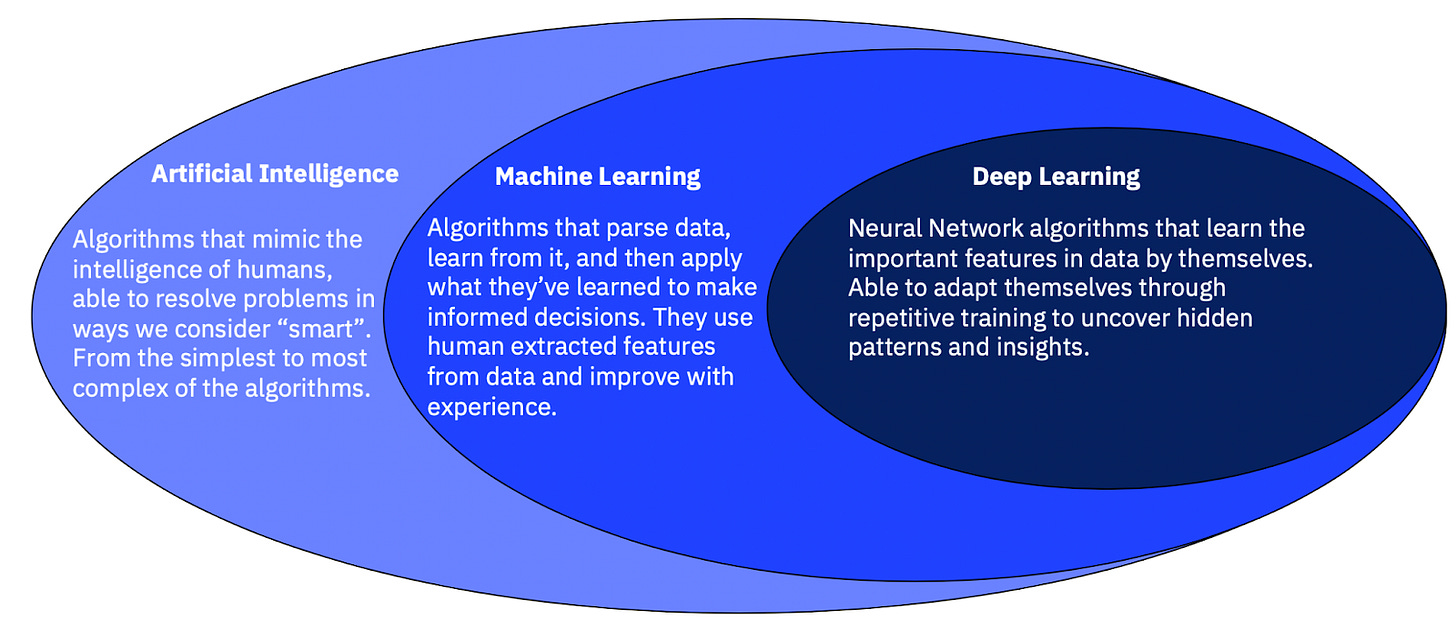
AI is split broadly into two groups: Artificial Narrow intelligence (ANI) and Artificial General Intelligence (AGI). When we talk about AI applications today, we’re talking about ANI, or “weak” AI, which are algorithms that can outperform humans in a very specific subset of tasks, like playing chess or folding proteins. AGI, or “strong” AI, refers to the ability of a machine to learn or understand anything that a human can. This is the stuff of movies, like the voice assistant Samantha in Her, the Agents in The Matrix, or Ava in Ex Machina.
Most of the things that we call AI today fit into the subset of AI known as machine learning. ML, according to Ben, is:
Code that is learned from data instead of written by humans. It’s inductive instead of deductive. Normally, a human writes code that takes data as input. ML takes data as input and lets the machine learn the code. In ML, algorithm = f(data).
ML has been around since the 1990s, but over the past decade, a subfield within ML called deep learning has ignited ML and AI application development. According to Andrew Ng, the founder of Coursera and Google Brain, deep learning uses brain simulations, called artificial neural networks, to “make learning algorithms much better and easier to use” and “make revolutionary advances in machine learning and AI.”
In a 2015 talk, Ng said that the revolutionary thing about deep learning is that it, “Is the first class of algorithms … that is scalable. Performance just keeps getting better as you feed them more data.”
Major improvements in ML and AI seem to come from step function changes in the amount of data a model can ingest.
In 2017, researchers at Google and the University of Toronto developed a new type of neural network called Transformers, which can be parallelized in ways that previous neural networks couldn’t, allowing them to handle significantly more data. Recent advancements in AI/ML like OpenAI’s GPT-3, which can write longform text given a prompt, or DeepMind’s AlphaFold 2, which solved the decades old protein-folding problem, use Transformers to do so. GPT-3 has the capacity for 175 billion machine learning parameters.
These advancements have led to a renaissance in the applications of AI and ML. I asked Twitter for some recent examples, and many of them are truly mind-blowing:

For all of the technological advancements, though, it all comes down to data. In his 2015 Extract Data Conference speech, Ng included this slide that highlights the benefit of deep learning:
What deep learning solved, and Transformers expanded on, is allowing models to continue to scale performance with more data. The question then becomes: how do you get more good data?
Getting to Scale
Scale has all the stuff that Silicon Valley darlings are made of: acronyms like AI, API, and YC, huge ambitions, young, brilliant college dropout founders, and an insight born of personal experience: AI needed more and better data.
Alexandr Wang was born in 1997 in Los Alamos, New Mexico, the son of two physicists at Los Alamos National Lab. Alexandr is spelled sans second “e” because his parents wanted his name to have eight letters for good luck. Whether through luck or genetics, Wang was gifted. He attended MIT, where he received a perfect 5.0 in a courseload full of demanding graduate coursework, before dropping out after freshman year. Wang worked at tech companies Addepar and Quora, and did a brief stint at Hudson River Trading.
In 2016, Wang (then 19) joined forces with Lucy Guo (then 21), a fellow college dropout (Carnegie Mellon) and a Thiel Fellow, and entered Y Combinator’s Spring 2016 batch. They didn’t quite know what they were going to build when they entered, but Wang, like so many founders, hit upon a problem through personal experience. He told Business of Business that at MIT:
There was nobody building anything with AI, despite the fact that there were hundreds of students at MIT, all brilliant, very hardworking people. We're all studying AI. And when I dug into it, I realized that the data was the big bottleneck for a lot of these people to build meaningful AI. It took a lot of time and resources to add intelligence to data, to make it usable for machine learning. There were no standardized tools or infrastructure, there was no AWS, or Stripe, or Twilio to solve this problem.
I even discovered it firsthand, because I wanted to build a camera inside my fridge, so it could tell me when to refill my groceries and what I need to buy. Even for that I just didn't have any of the data to make it work.
So Wang and Guo built Scale during YC, and launched it at the end of the program, in June 2016. Before it was Scale AI, they called it Scale API.
If Scale’s ambitions were as large then as they are now, the co-founders hid that ambitious light under a bushel. At the time, the value prop they pitched was clear: API for Human Tasks.
While there was a lot of hype around what AI might do, the fact remained that there were many tasks, even repetitive and seemingly-simple ones, for which humans were much better suited. On the first version of the website, uncovered by the Internet Archive’s Wayback Machine, Scale listed three examples:
At this point, Scale billed itself as a more reliable, high-quality version of Amazon’s Mechanical Turk, which used APIs to simplify the process of requesting work, and vetted, trained people on the backend, with a peer-review system, to ensure quality outputs. Instead of hiring internal teams of people to review content on a social media site, for example, a company could feed content through Scale’s API to teams of people trained to decide whether something someone wrote was against the site’s terms of service.
This 2016 Software Daily podcast with Wang and Guo was a good representation of the way they described the business then:
In that interview, they hinted that the product had moved beyond just content moderation and data extraction to what would become their calling card: data annotation. That same month, image recognition showed up on the website as a use case for the first time.
For the first year, Scale seems to have survived on the $120k that YC invests in exchange for 7% of the company. Then, in July 2017, it announced a $4.5 million Series A led by Accel.
Scale’s Evolution as Told Through Funding Announcements
Funding is not the most important thing for a startup, but the history of Scale’s funding announcements tell the story of the evolution in its focus and the way it described itself.
Already, in the Series A announcement, Scale unveiled its larger ambitions, not just a better Mechanical Turk, but a better API for training data. The announcement focused squarely on AI, mentioning it seven times:
Our customers agree that integrating AI with accurate human intelligence is crucial to building reliable AI technology. As a result, we believe Scale will be a foundation for the next wave of development in AI.
If the 2017 Series A highlighted the evolution from API to AI (it would change its website from scaleapi.com to scale.ai in February 2018), the August 2018 announcement of Scale’s $18 million, Index-led Series B was about its role in data labeling for autonomous driving. The post highlighted that:
In autonomous driving, one of the most prominent applications of deep learning today, Scale has become the industry standard for labeling data. We’ve partnered with many industry leaders such as GM, Cruise, Lyft, Zoox, and nuTonomy. We’ve labeled more than 200,000 miles of self-driving data (about the distance to the moon).
At this point, it’s worth pausing to describe just what data labeling is.
Data Labeling: Hot Dog, Not Hot Dog
As we covered above, ML models take data as an input and let the machine learn its way into figuring out the right code. Without data, there is no ML or AI, and bad or mislabeled data is worse than no data at all. “Garbage in, garbage out.”
To get data to feed the models, companies can either use open data sets, buy data, or generate the data themselves. Scale’s largest customer segment to date, companies building autonomous driving technology, generate petabytes of data as they drive around and capture video and Lidar point clouds of their surroundings. In their raw form, those videos don’t mean anything to the models that make decisions about when to stop, go, swerve, speed up, or slow down. So companies like Toyota and Lyft send huge files to Scale, and Scale’s job is to send the files back labeled, or annotated.
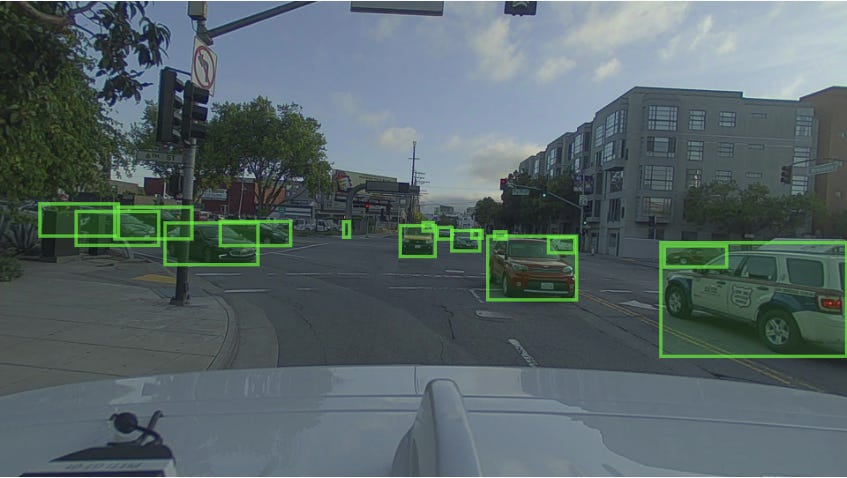
Scale’s system is a “Human-in-the-Loop” (HIL) system. From a cost and speed perspective, the ideal would be to have algorithms tag everything, but the algorithms aren’t good enough yet (and certainly weren’t good enough when Scale launched in 2016). This scene from Silicon Valley captures it well:
So instead of relying on the machines, Scale’s algorithms break the videos into frames, take a first pass at labeling the images, and loop in humans for the trickier ones. Humans then label the unclear parts, and send labeled data to Scale, which checks it with more ML and human reviewers and sends it back to customers, who use the annotated data in their models. This process should be familiar to everyone reading this: every time you’ve had to “Select all images with boats” to log into a website, you’ve been labeling data to train models.
On the data labeling front, the goal for Scale over time is to increase the percentage of images that it can label without humans. That drives down cost and improves margins. Importantly, as humans label images, they’re also training Scale’s algorithms, and making them more accurate over time. This is Scale’s data flywheel.
Now we’re ready to go back to Scale’s evolution as told through funding announcements.
Scale’s Evolution as Told Through Funding Announcements, Part Deux
In August 2019, Scale announced a $100 million Series C, led by Peter Thiel at Founders Fund, with participation from Accel, Coatue Management, Index Ventures, Spark Capital, Thrive Capital, Instagram founders Kevin Systrom and Mike Krieger and Quora CEO Adam d’Angelo. Scale became a unicorn in this round, with a $1 billion valuation on the nose. TechCrunch highlighted the diversity in its customer base, no longer just AV, but “OpenAI, Airbnb and Lyft,” as well. Thiel pointed out Scale’s strategic positioning as an infrastructure company:
AI companies will come and go as they compete to find the most effective applications of machine learning. Scale AI will last over time because it provides core infrastructure to the most important players in the space.
This is an important piece: as an infrastructure company with a diverse customer base, Scale’s success isn’t indexed to the success of any one company, industry, or use case. Instead, Scale succeeds if AI and ML usage grows more broadly. An AV-data-labeling company lives and dies with the rise of AVs and their need for fresh, clean data; an AI infrastructure company is positioned to succeed no matter what unpredictable applications of the technology flourish.
Meanwhile, Scale kept growing. In Q3 2020, just four years in, Scale hit a $100 million ARR run rate, making it one of the fastest companies ever to reach that milestone.
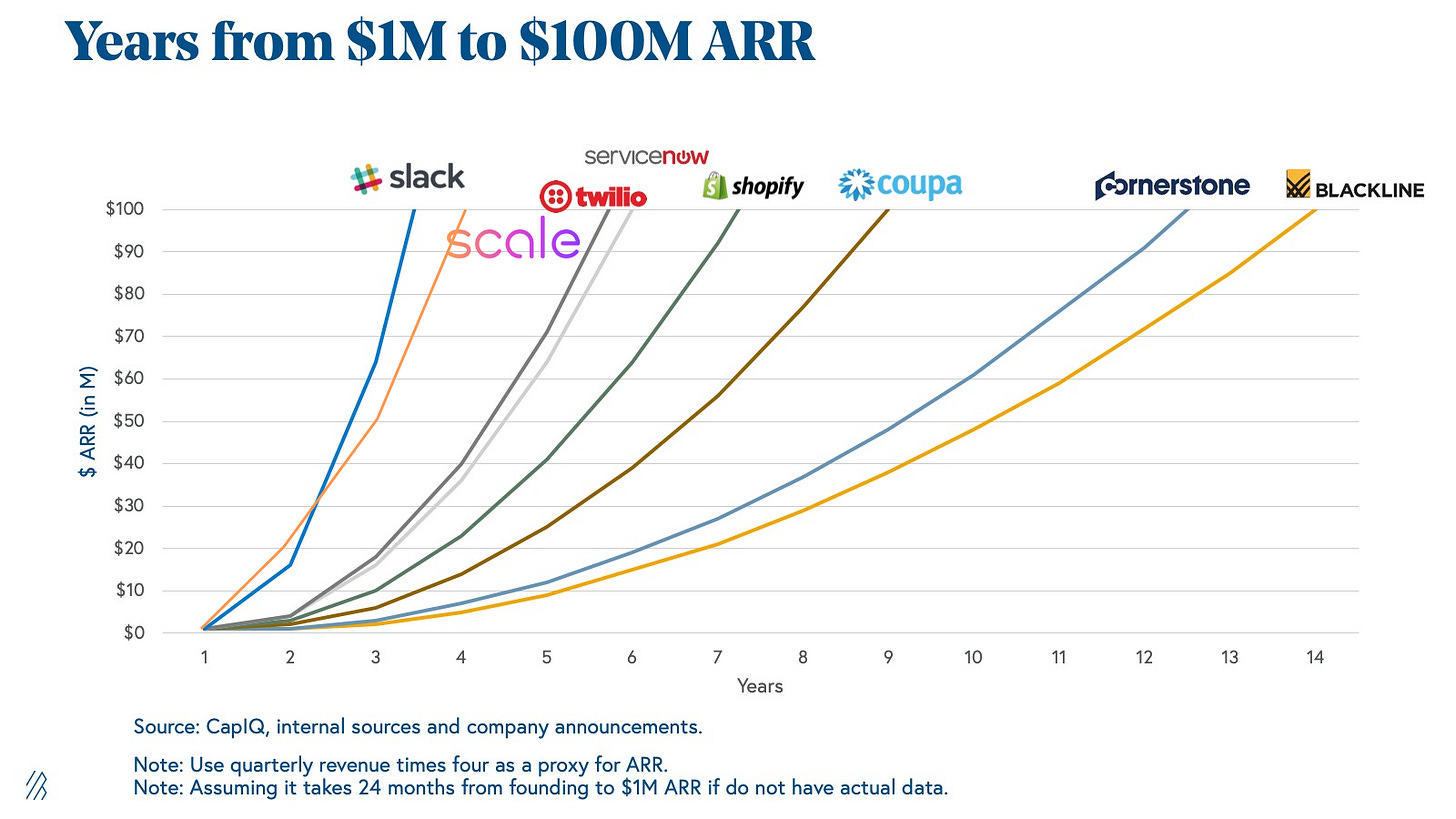
An orange line like that is Tiger bait, and indeed, Tiger Global came calling, leading the company’s $155 million Series D at a post-money valuation over $3.5 billion. In the announcement on its blog, Scale highlighted its expansion beyond labeling with Nucleus:
Our team is tackling the next bottleneck in AI development: managing the full life cycle of AI development across teams. Building and deploying scalable machine learning infrastructure is slow, manual and costly, and in August we launched Nucleus to enable seamless collaboration across the AI development cycle.
Nucleus, which Wang described to me as a “data debugging SaaS product,” helps companies visually manage and improve their own data, and drives faster cycle times in annotation using software. According to Scale’s website, “Nucleus provides advanced tooling for understanding, visualizing, curating, and collaborating on your data – allowing teams to build better ML models via a powerful interface and APIs.” It’s like Google Photos meets Figma for your data.
Visualizing and collaborating on data lets teams go through the ML lifecycle more quickly, and going through the ML lifecycle more quickly lets companies develop better models. Scale’s bet is that companies will be able to go through the lifecycle more quickly if they keep everything inside of Scale.
Nucleus also provides a cheaper, lighter-weight, self-serve solution for Scale to begin to capture the long-tail of customers and deploy AI across every industry.
In fact, Deploying AI Across Every Industry was the title of the announcement post for Scale’s recent $325 million Series E, led by Greenoaks Capital, Dragoneer, and Tiger Global, which valued the company at $7.3 billion. (This is the round I participated in.) The announcement highlighted Scale’s ambitions to allow companies to manage the full ML lifecycle:
At Scale, we’re building the foundation to enable organizations to manage the entire AI lifecycle. Whether they have an AI team in-house or need a fully managed models-as-a-service approach, we partner with our customers to build their strategy from the ground up and ensure they have the infrastructure in place to systematically deliver highly-performant models.
No longer just a data labeler for AV companies and tech giants with in-house AI and data science teams, Scale is beginning to manage more of the process, enabling companies that might not otherwise have in-house teams to build products using AI. In the announcement, Scale highlights that companies like Brex and Flexport use its Document product, which it launched in April 2020 -- Brex for invoices, and Flexport for logistics paperwork. Document is significant because Scale not only labels data, but works with customers to build custom models. Brex doesn’t have an AI team working on the model; they outsource it to Scale.
Brex CEO Henrique Dubugras told me that they use Scale to build a one-click bill pay product that takes an invoice, extracts the data, and lets the recipient pay in one click. But even though Scale has been a Brex customer forever, Brex actually didn’t turn to Scale first. It turned to a bunch of companies that specifically do Optical Character Recognition (OCR) and invoice extraction, but said “they were all mediocre.” When Brex turned to Scale, Dubugras said that they worked together to build and improve the model.
“Not only was the extraction rate of the ML model better,” he said, “the integration between the ML models and humans was special. You need accuracy for a one-click invoice pay, and putting humans in the loop helped us trust the accuracy.” With specifically-built invoice extraction solutions, the accuracy was in the 70% range, but using Scale, “with humans, we get very close to 100%.” It’s a proof point that by starting with data labeling, Scale can do more than data labeling.
The early success and adoption of Nucleus and Document are beginning to prove out Wang’s thesis: that data is the fundamental building block on top of which to build out a complete product offering. If that strategy sounds familiar, that’s because it is.
Scale has a lot of similarities to Stripe…
Scaling Like Stripe
Everyone likes to compare themselves to Stripe. How could they not? Stripe is almost universally beloved, by engineers and investors alike, and has built a $95 billion payments infrastructure company in just over a decade.
If a company is API-first or writes clean documentation, it’s going to draw comparisons to Stripe, either from outsiders or from the company itself. But APIs and documentation are only two chains in a larger chain-link strategy that makes Stripe what it is.
Zooming out, Stripe’s strategy was to set a lofty mission -- Increase the GDP of the Internet -- and start by spending years perfecting the fundamental building block: ecommerce payments on the internet. If that building block looks like a commodity, all the better. That means less competition from more sophisticated players until it’s too late.
Stripe obsessed over payments from the earliest days. They were convinced it was the fundamental building block, the atomic unit of the internet economy. In Stripe: The Internet’s Most Undervalued Company, I wrote about Stripe’s evolution:
In a decade, Stripe has gone from accepting payments, which is now a commodity business, to providing an increasingly comprehensive suite of products that make it easy to start and run an online business.
Stripe took its time building out the comprehensive suite. Mario Gabriele recently wrote an excellent deep dive on the company called, Stripe: Thinking Like a Civilization, in which he included a striking timeline:
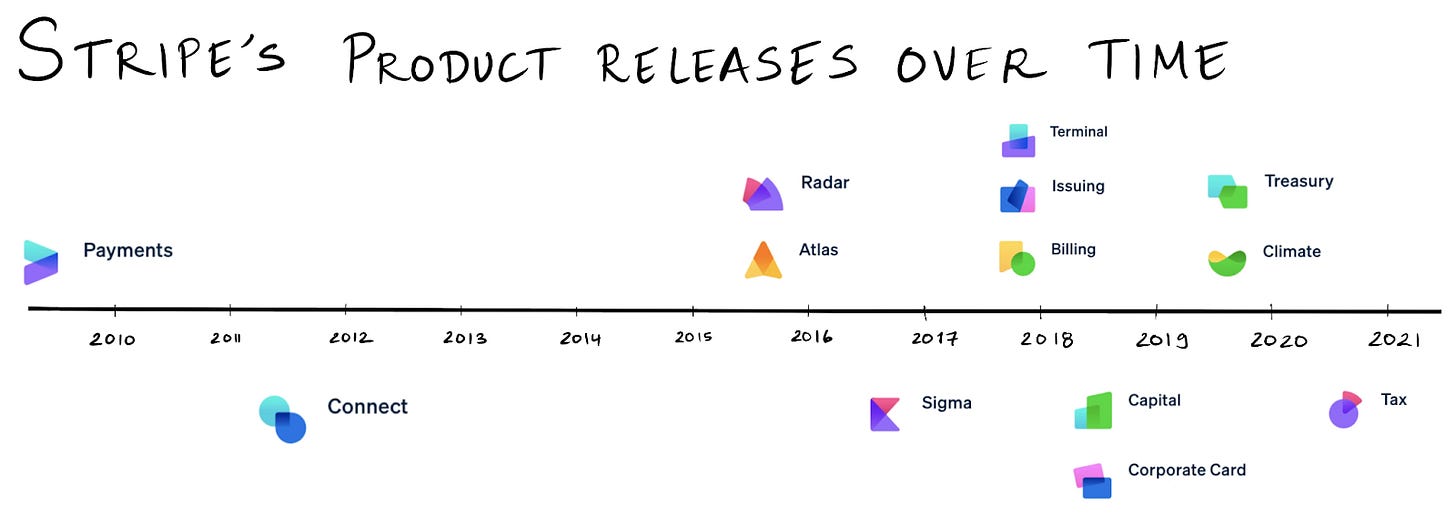
Stripe built its Payments APIs, the core technology on top of which everything else was built, in the very beginning, 2009. It spent years perfecting it, with only one other new product in the first six years of its life: Stripe Connect. It launched Connect, which lets marketplace businesses pay out the supply side, by working hand-in-hand with Lyft, one of its biggest customers at the time. And then it went dark, entering a four-year period in which it released no new products.
Stripe didn’t get lazy; it was like a coiling spring. In 2015, Stripe CEO Patrick Collison told Stanford’s Blitzscaling class, “When you come out of [that period], if you do it well, then stand back.”
Stand back, indeed. Since 2015, Stripe has launched 13 new products. Mario published eight days ago. Since then, Stripe rolled out two new ones: Identity, online identity verification, and Terminal, its first hardware product. As Ben Thompson points out, all thirteen products (except for Identity) were built on top of Stripe’s Payments engine, that little piece of supposedly commoditized infrastructure it built more than eleven years ago.
Scale’s bet, and its investors’, is that labeled data is to the development of AI applications what payments is to increasing the GDP of the internet. The view might be: “If there is a Stripe for AI/ML, it would look like Scale. Just like Stripe was able to leverage the Payments foundation, Scale will grow downstream by leveraging annotated data.”
Scale spent its first four years getting really, really good at data labeling and annotation, driven by the belief that data is the fundamental building block of AI and ML. It’s the default choice for AV companies, one of the hardest categories to serve because quality is a matter of life and death, and has expanded to other industries including government, healthcare, and tech.
Like Stripe, it went into a four-year quiet period. Now, it’s starting to ship products across the ML lifecycle: Annotate, Manage, Automate, Evaluate, Collect, Generate.
Annotate: This is Scale’s bread and butter. Its focus here is to increase the proportion of annotation work done by algorithms instead of humans while maintaining quality. It just rolled out (hours after sending this) a new mapping product to expand its strength in annotation to help companies create accurate, custom maps.
Manage: Scale launched Nucleus in August 2020. Nucleus lets companies manage, curate, and understand their data through a visual web-based interface, and brings the process to the cloud. Nucleus lets Scale serve smaller clients in a more self-serve way.
Automate: Scale released Document in April 2020. Document uses Scale-built machine learning models to process and extract data from documents. It’s Scale’s first product that gives customers the output -- trained models -- rather than just the input -- labeled data -- and could replace the need for AI/ML teams entirely in some cases.
Evaluate: Nucleus also lets customers test, validate, and debug models. They can upload predictions via API, track model performance over time, compare runs, sort failure examples by metric, and build model unit tests on curated slices of data.
Collect: Scale’s homepage currently has a waitlist for a new product that will help companies collect data. It highlights the ability for companies to gather text and audio data in 50+ languages from 70+ countries.
Generate: It also has a waitlist for a product that would generate synthetic data to “expose your model to more data than you could otherwise collect.”
Scale is coming out of that period; stand back.
These things look obvious in hindsight, but not in real-time. Greenoaks is one of the best venture funds in the world, returning 51% annually since 2012, and it had evaluated Scale in the past, but didn’t invest until co-leading the Series E. In the interim, Scale kept executing on its vision and continued to add proof points to support its thesis. By the time the opportunity to lead the Series E came around, Greenoaks’ Neil Mehta was a believer. He explained his epiphany to me: “I went from thinking data annotation was a commodity service, to ‘Data annotation is the source of truth.’ If you don’t annotate data correctly, nothing else works.”
Annotated data and payments share another advantage: they’re both at the top of the funnel. In Stripe’s case, fraud detection, tax collection, marketplace payouts, and the other products don’t happen without a business first getting paid. Stripe can catch a company at its earliest point, and grow with them. In Scale’s case, the ML lifecycle starts with good data; you can’t manage, automate, or evaluate without it. Scale believes that, like Stripe, it will be able to acquire customers at the top of the funnel, and sell them a connected suite of products over time that work together to create faster cycle times, which create more accurate models, and better outcomes. Because it’s starting with the piece that looks like a commodity, it faces less competition from the big players in the space, whose AI teams are too valuable to waste on annotation. That’s its wedge.
That said, to be truly Stripe-like in its growth, Scale will need to release products to better serve the long-tail of smaller customers, those with engineering teams but maybe not AI/ML teams, as it’s begun to do with Brex and Flexport. There seems to be a pattern to its product development: human-heavy first, software-heavy over time. My hunch is that it’s working hand-in-hand with companies like Brex and Flexport to develop custom solutions that it will ultimately roll out off-the-shelf as APIs or SaaS as the models, and the data that feeds them, get good enough.
When it does roll out more self-serve products for engineers, the demand will be there. Stripe and Scale seem to share one more important quality: developer love. Dubugras told me that when he told the Brex engineering team they were working with Scale, the engineers got super excited. I asked why, and he cited a few reasons: “The APIs are so good, they have open source data sets, the documentation is clean, they hire the absolute best engineers, and they get the small details right. Scale has a Stripe-like halo effect with developers.”
For both Stripe and Scale, companies that slowly built up a head of steam before outbursts in productivity, it’s all about slope over intercept. Start slowly with a commodity product, get it right, hit an inflection point, and shoot up as fast as you can to leverage your strategic place in the value chain before anyone else can match you from their seemingly differentiated starting point.
And speaking of slope, from a valuation perspective, Scale is actually outpacing Stripe in terms of valuation. It was valued at $7.3 billion 4.9 years into its life, while Stripe was valued at $3.5 billion at the same point. Stripe didn’t break $7 billion until it was valued at $9 billion 6.9 years into its life. (Obviously, we are in a very different and pricier market now than we were then.)
Despite its growth across product, revenue, and valuation, though, there are many who still believe that Scale is an overvalued human-powered commodity services business. Let’s look at the bear case.
The Bear Case for Scale
I’m a sucker for a good long-term strategy. My brain naturally searches for confirmatory evidence. “Aha!” it says, “A successful new product launch, the master plan is working!”
To guard against that, I went in search of opposing viewpoints, from friends in the industry and in Tegus transcripts. Synthesizing all of the knocks and critiques, this is the bear case for Scale:
Data labeling is a commodity, and it’s not a critical one. Over time, Scale’s human-heavy approach will become obsolete since more data will be auto-labeled, more human labeling will be done by mobile gig workers, and unsupervised learning models, which don’t require labeled data, are taking off anyway. Plus, these models might not be as generalizable as Scale needs them to be, so it will be harder to move downstream than anticipated. On top of all of that, Scale faces competition from above and below -- the biggest tech companies in the world are pouring tons of resources into AI/ML and new startups are springing up every day.
When I went in search for a bear case for Stripe, I wrote that, “It’s hard to take a Stripe bear case too seriously, because there is no way that the Collisons haven’t thought much more deeply about the challenges it faces than anyone else in the world.”
There’s a similarly reductive reason that it’s hard to take Scale bears too seriously: the company’s slope is so steep that many of the critiques from ex-employees and others in the industry seem to be out of date even if their information is just a few months old.
Scale faces challenges, to be sure, but it’s knocking them down at a rapid clip.
Let’s take them one-by-one.
Data labeling is a commodity, and it’s not a critical one.
There are two pieces to this: whether data labeling is a commodity, and whether it’s critical.
First, while hot-dog / not-hot-dog style labeling is now a commodity, Scale’s customers demand extremely high-quality, very fast turnaround times, the ability to handle large volumes, or all of the above. One way to test whether it’s a commodity is whether Scale has pricing power. Based on conversations and Tegus transcripts, it does seem to be able to command higher prices than competitors in exchange for meeting tighter SLAs.
Second, data is critical. As discussed, the biggest advances in the industry have come from enabling models to ingest more data. Andrew Ng, a leader in the field, is on a crusade to give data its due.

The industry’s underappreciation of data is Scale’s opportunity. Many believed payments to be a commodity, and that was to Stripe’s advantage. The longer Scale can build up its data advantage while others believe it’s a commodity, the harder it will be for anyone else to catch up.
Scale’s human-heavy approach will become obsolete.
Scale would agree that a human-heavy approach isn’t the right one in the long-term, but it’s crucial to the data flywheel. As Scale’s human teams label data, they’re also training Scale’s labeling models. Over time, the ratio of human-to-machine has decreased; more work is being done by the algorithms. As a simple way to think about it, consider how hard Captchas have gotten versus how easy they used to be. Humans almost need a PhD or 20/10 vision to pass a Captcha these days. Algorithms can do more and more of the work, leaving humans with the more challenging jobs and edge cases.
The move to more algorithmic tagging is actually a boon for Scale, which has trained its models on more human labels than nearly anyone in the world. It’s much worse for competitors like Appen, which are more akin to Upwork-for-labelers than an AI company.
These models might not be as generalizable as Scale hopes or thinks.
For Scale’s grand plan to work fully, it needs to be able to apply a scale and data advantage to build models that work across a wide range of use cases and perform better than models developed by companies that focus on particular use cases. While Scale might have an advantage building computer vision models for AVs, this argument goes, the same won’t be true in different areas, like Natural Language Processing (NLP). In a Tegus interview, one former Scale account executive expressed doubt that Scale could compete with Appen for NLP deals, for example. He said that since Appen had historically focused on NLP and Scale had historically focused on computer vision, it was hard for either to win business in the other’s turf.
That interview is less than a year old, and already it seems a bit stale. It’s still early days for Scale in terms of productized model development, and Brex and Flexport are just two examples, but I thought a point Brex’s Dubugras made was telling. He said that when Brex started using Scale Document, it didn’t have the exact models Brex needed to extract and action data from invoices, but that it got really good, really fast. Slope over intercept.
“It took a little time to train the model,” Dubugras explained, “But once it was trained, it was a lot better than anything else we tried.” Scale wasn’t known for OCR and NLP, but it trained the model on the right data, and within weeks, had the best product of any that Brex had tried. Speaking of commodity products, he told me “I didn’t think that OCR would be a competitive differentiator, but the OCR was so good that our reps actually sell ‘OCR that actually works’ as part of the value prop.”
Certainly, Scale has a lot to prove as it tries to expand its capabilities across the lifecycle and across use cases, but early results have been good, and they’ve been good because of good data.
Scale faces competition from above and below.
There is a data and AI arms race afoot. Every year, FirstMark’s Matt Turck puts out a report on the data and AI landscape, in which he includes a market map. This is the map from the 2020 Report:
Scale is that tiny little red circle in the “Data Generation & Labeling” category. Just in its own category, it faces competition from Amazon’s Mechanical Turk, which provides much cheaper but lower-quality labeling, as well as Hive, Appen, Upwork, Unity, Lionbridge, Labelbox, and AI.Reverie. Still, Scale seems to be the leader in the category, but even so, Data Labeling & Generation is just one of twenty categories within the “Infrastructure” segment. Just looking at the market map, it’s overwhelming to think about how Scale might emerge from its tiny box to become the infrastructure layer for AI & ML.
Additionally, the biggest tech companies in the world -- Facebook, Microsoft, Google, and more -- are pouring billions of dollars into AI research. Facebook has a content moderation team of over 10,000 humans tasked with removing bad content from the site; it’s incentivized to figure out how to remove humans from the process. In her Substack in mid-May, Google ML PM Aishwarya Nagarajan showcased Facebook’s DINO, its new computer vision system that can segment images without any training data:

Even scarier, Facebook open sourced the DINO code and pre-trained model.
To me, a non-AI researcher, non-ML engineer, non-data scientist, it would seem that circumventing the need for labels in the first place would neutralize Scale’s advantage in data labeling. This seemed like the most legitimate bear case to me, so I asked Wang whether self-supervised learning was an existential threat to Scale. His two-part answer made me even more bullish:
Self-supervision doesn’t remove the need for human-labeled data, it just shifts it. The common view of top researchers is that self-supervision will produce a model with reasonable “base” capabilities, but that to create an algorithm that’s useful in the real world, you need labeled data for a process called “fine-tuning.” Even the top researchers in self-supervised learning are actively researching how to use labeled datasets to get models to perform better. See: OpenAI, here, here, and here.
Deflationary technology actually increases market sizes, particularly in strategically valuable industries like AI. Most techniques in research aim to increase efficiency in the use of labeled data -- say getting the same result from 50 labeled data points instead of 100. Instead of decreasing demand, more efficiency might create more demand for labeled data. As a comparison, Moore’s Law has improved the efficiency of compute infrastructure by 2x every two years. That has exploded demand for chips, as more efficiency allows for new applications, which increase aggregate demand. Wang believes that a similar phenomenon will play out in AI, accelerating the growth of the market.
When even seeming bear cases are actually tailwinds, you’re in the right market. Like Ethereum, given Scale’s place in the value chain, as the infrastructure layer, it stands to benefit from increased competition in the space. More AI and ML activity is net good for Scale. The question now is just how much of the opportunity Scale can capture.
The Bull Case for Scale
For such a complex space and long-term focused plan, the bull case for Scale is simple:
Scale is growing incredibly fast in an incredibly fast-growing, nascent market while proving out its ability to successfully expand into new industry verticals and new product lines by leveraging data as the foundational building block. Its data flywheel spins faster and faster. If it’s successful, Scale will be the infrastructure for a space that grows to touch every sector of the economy, and will compound like the leading infrastructure companies do.
Let’s break it down.
Scale is growing
First things first, Scale is growing fast. It more than doubled revenue last year to over $100 million, and it did so by growing much faster with customers outside of the AV space, from the Department of Defense to PayPal.
At the same time, its thesis around HIL systems is coming true: Scale has been able to lower prices and increase margins as software learned from its human partners and can handle a higher proportion of labeling. Scale has expanded margins dramatically over the past two years, even as it’s lowered prices. It looks a lot more like a software business than a services business now.
While Scale is growing, the company and the industry more broadly are still in their infancy. Some stats paint the picture:
The majority of the world’s data has only been created in the last two years.
Only 8% of data professionals believe AI is being used across their organizations, and nearly 90% of employees believe data quality issues are the reason their organizations have failed to successfully implement AI and machine learning.
MIT Sloan Management Review found that only 7% of organizations had put an AI model into production.
There won’t be an industry that data-trained models won’t impact in the coming years -- from healthcare to manufacturing to finance to security to transportation -- and if Scale’s thesis continues to play out, it should be able to serve each of them across the full range of AI/ML infrastructure. That represents an enormous opportunity.
The AI infrastructure market is expected to reach anywhere between $100-300 billion in the next few years, and it’s growing fast. Just looking at the products it offers today, Scale expects the addressable market in data annotation to exceed $20 billion by 2024, and it sees the addressable markets for Document and Nucleus to reach $10 billion and $8 billion, respectively, by the same year. Before accounting for new products with which it’s experimenting, Scale is directly attacking a $40 billion addressable market.
Being the infrastructure provider in a fast-growing, rapidly evolving market is an indexed way to capture value: no matter which technologies, applications, or companies emerge victorious, Scale benefits from their competition and efforts to grow.
Expanding into New Verticals and Product Lines
With each passing month, Scale de-risks its thesis. It seems increasingly true that annotated data is the source of truth and the fuel for the growth of AI and ML, and Scale is rapidly proving out that it’s able to annotate all sorts of data. It found its original niche in labeling data for AV’s computer vision models -- cars, stop lights, stop signs, etc… -- but it’s increasingly proving two things:
It’s good at labeling all sorts of data. Its customers include government agencies like the DoD, marketplaces like Airbnb, fintech companies like Brex, and even AI powerhouse and GPT-3-developer OpenAI. Each has very different data labeling needs, but Scale has proven that it is able to win contracts and deliver quality service to each of them.
Data defines the systems. As it expands verticals, it also expands its product offerings, leveraging its data advantages. It’s proving early success in building models across verticals, and proving out what Wang told me when we first spoke: “If you have one algorithm on a self-driving car that recognizes pedestrians, and another that detects tumors, the code is actually very similar. The difference is the data.”
Document is an incredibly important early proof point, and one that dramatically raises Scale’s ceiling, from the world’s best data annotation company used by AI teams to infrastructure for AI development used by any company that might want to incorporate AI/ML.
Data Flywheel
If Scale becomes a $100 billion company in the next few years, its data flywheel will most likely be the reason. Scale benefits from a standard marketplace flywheel:
More customers → more labelers → faster turnaround and higher throughput → more customers.
But Scale also benefits from data network effects that spin the flywheel faster than that, because as more labelers label more customers’ data more quickly, Scale’s models also improve, which spins the whole thing more quickly.
If better annotated data is the key building block for the rest of the AI/ML infrastructure layer, if it’s the atomic unit of AI/ML that makes everything downstream better, then Scale’s data flywheel gives it an unfair advantage up and down the stack.
Moats
Taking an early lead is one thing; protecting profits against well-funded competitors is another. To protect profits, you need moats, and to analyze moats, you need to turn to 7 Powers.
I think Scale benefits mainly from three Powers: Scale Economies, Switching Costs, and Cornered Resources.
Scale Economies. This is the data flywheel. The more customers Scale has, the better its models get, and the less it costs Scale to label data at the same level of quality. I was close to calling this a data network effect -- it gets more valuable for every customer when each new customer joins -- but scale economies is more accurate. This should extend beyond just data labeling and across products. For example, the more customers who use Document and the more data Scale trains the models on (with human input to start), the better the models will get at handling all sorts of documents. Scale should also be able to lower its cost to deliver models over time.
Switching Costs. Switching costs are fairly weak in Scale’s Annotation offerings alone -- it keeps clients by having a higher-quality product, but it’s not particularly hard to switch -- but with Document and future model products, it will become increasingly difficult for customers to switch off of Scale. That’s because Scale continues to improve its customers’ models with usage, and they’d need to retrain the model if they switched. Dubugras said as much, “If we moved away from Scale, we’d have to restart from scratch.” Plus, he said, switching off of Scale would also mean “a fight with my engineers, which I’m not interested in.”
Cornered Resources. Scale arguably has a cornered resource in the data and models that it’s built over the last five years, but its real cornered resource is AI talent. When I asked Dubugras why Brex wouldn’t just hire a team to build the models themselves, he revealed one of Scale’s biggest advantages: “Look, they have a lot of the best AI talent in the market. I can’t just snap my fingers and hire a great team. AI engineers want to work at the companies solving the hardest AI problems, and Scale is one of them.” By cornering the market on great AI talent, Scale can build better products for customers and make it infeasible for most customers to try to bring the capabilities in-house.
I could make an argument for Process Power (human-in-the-loop labeling that gets more efficient over time), Brand (particularly among developers), and Network Effects (as described above), but Scale Economies, Switching Costs, and Cornered Resources are the big three for Scale. They each feed into each other and dig moats around the business, setting Scale up to compound for a long time.
The Compounding Vision: Rationality in the Fullness of Time
Everything in the bull case compounds. It spins faster and faster in the present towards an unimaginably huge vision for the future.
When we spoke, Wang told me that he looks to Amazon as the best example of how to build a company, pointing out that “Amazon is interesting because they operate on both really long and really short timelines.” That’s a tough balance to strike, but Wang is getting guidance from someone who would know how to do it: former Amazon exec Jeff Wilke. It seems to be pulling off the balance.
In the short-term, it’s growing fast by building in the direction of its mission while being flexible enough to build based on its clients’ needs. Just like Stripe Connect was an ask from Lyft, Scale is willing to roll up its sleeve and work with clients to build custom solutions, like it did with Brex.
That means more revenue today, which means more funding, which means more talent, which means better product, which means more customers, which means more and better data, which means better models, which means higher margins and faster cycles, which keeps the whole flywheel spinning and spinning.
In the long-term, Scale is building a potentially unimpeachable position as the source of truth in a world that will increasingly depend on clean data. From that beachhead, it’s expanding into new product lines, augmenting AI and ML teams at large tech companies, and bringing the full range of capabilities to companies that couldn’t build with AI and ML on their own, but need to in order to survive.
“If you can provide the infrastructure to support major categories,” Wang explained, “the thing that’s unique is that you can compound for a long time. Look at Twilio, Stripe, and Snowflake. If Scale is the critical platform for much of AI work, it’s massive. It’s arguably bigger than all of the other ones.”
That’s the thing about compounding: it takes time, and then it rips. If all of that grows out of a commodity data labeling business, we’ll only be able to appreciate its brilliance in the fullness of time.
Thanks to Dan for editing, and to Alex, Ben, Neil, Henrique and others for the input.
How did you like this week’s Not Boring? Your feedback helps me make this great.
Loved | Great | Good | Meh | Bad
Thanks for reading and see you on Thursday,
Packy
This is like "promoted content" on Outbrain. Please stop the shilling and provide original insights.
very interesting. would be informative to see what their seed pitch looked like.