Unit21: No-Code, Much Power
Meet the $300M Tiger-Backed Startup Empowering Operators One Vertical at a Time
Welcome to the 1,133 newly Not Boring people who have joined us since last Monday! Join 59,956 smart, curious folks by subscribing here:
🎧 To get this essay straight in your ears: listen on Spotify or Apple Podcasts (soon)
Today’s Not Boring, the whole thing, is brought to you by… Unit21
Unit21 gives operators and analysts powerful activity monitoring tools that they can customize to their needs — from AML to Identity Verification and even to Recruiting and Lead Generation — all without writing any code. They’re well-funded and hiring, join them!
Hi friends 👋 ,
Happy Thursday! After a week off for the 4th of July and a move back to Brooklyn, we are back!
Today’s post is a Sponsored Deep Dive — that means they’re paying me — on a fast-growing startup that just yesterday announced a $34 million Series B at a $300 million valuation led by Tiger Global.
This essay is a great example of what I want Sponsored Deep Dives to be: a behind-the-scenes look into a fast-growing, under-analyzed startup’s philosophy, strategy, and tactics, chock full of lessons for both builders and investors. Plus, there’s an added bonus: a glimpse into how a Tiger-led round comes together (spoiler: quickly and ferociously).
The company announced the raise in TechCrunch yesterday. That gave the “what.” Today’s piece gives the “how and why.”
Let’s get to it.
Unit21: No-Code, Much Power
When my friend Prince Boucher reached out to me in April about the company he’d recently joined, Unit21, I was excited… to catch up with Prince. I’d never heard of Unit21. He explained what they did -- no-code/low-code tools to fight financial fraud -- and asked if I’d be interested in writing about them. If I’m being honest, I was only quasi-interested. To be sure, it’s an important topic -- financial fraud has increased as commerce moved online during COVID, crypto presents new challenges, the Biden Administration is supportive of regulatory tech (“RegTech”) solutions -- but most of you don’t work in risk, compliance, or RegTech. I wasn’t sure if this was something you might be interested in.
I told him I’d do a little research and get back to him.
Then I did my research. Unit21 isn’t just a KYC/AML tool for financial institutions. That’s just the first product, an appetizer, the white hot core from which it will expand outward. What Unit21 is really building is a business logic, flagging, and reviewing engine that lets non-technical people run rules on constant streams of data. More simply, Unit21 lets ops people get shit done at scale without going through engineers. It lets companies empower their people and focus resources on the right things.
My interest was piqued, then serendipity sealed the deal. When I was researching my essay on Scale, I had already spoken with Unit21’s co-founders, Trisha Kothari and Clarence Chio about their vision, so this exchange from a Tegus interview with a former Scale VP hit me in the face:
Question:
So what are the trends or like who should I most pay attention to if AI is the next big thing?
Answer:
What I would actually look at, if I were you, is to look at what's emerging as the low-code/no-code market…
So what's happening is there's a bunch of start-ups that are emerging that are doing basically offering AI and ML via kind of a low-code/no-code way, meaning that if you're a business analyst, and you don't have necessarily coding experience, but you understand just computing in general, you're still able to create models like they're doing this industries-wise, right?
So there's a lot of work happening in the financial services industry, for example, for the biggest problems like fraud detection, for risk management, for like basically threat to skimming money and all those use cases that have been problems in the financial services industry, for example. We have solutions to kind of solve it through ML and AI.
I would wager a significant sum that he was referring to Unit21. He highlighted both its current offering and its vision.
Fraud detection in financial services is where Unit21 has made a name for itself, but what Unit21 is really building is “basically offering AI and ML via kind of a low-code/no-code way.”
Low-code/no-code doesn’t mean that Unit21 doesn’t write code -- on the contrary, the team is solving some cutting edge machine learning and engineering problems. It takes high-code to deliver no-code. Unit21’s team handles all of the complexity below the surface and delivers it through a simple API and dashboard so that operators and analysts can customize it to their needs without having to write code themselves.
So yes, even if you don’t work in risk or compliance or finance at all, it’s certainly worth learning about, for a few reasons:
A novel approach to building out a platform and striking a delicate balance between power and flexibility, generalizability and localizability.
Getting AI/ML tooling into the hands of non-technical users.
How no-code/low-code may be more important than I realized on the B2B side.
An exclusive look at how Tiger Global decides to invest. 🐅 👀
Unit21 is fascinating both for what it’s building and for how it’s building.
Instead of starting with a generalizable platform, Unit21 built a tool for the financial fraud vertical and is now expanding outward to evermore use cases. The dream end state is a product that feels like it was custom built for each company’s needs even though it was built to flexibly handle all of them. Clarence described it as similar to Amazon’s recommendation engine, for business.
The approach is working. Major companies like Coinbase, Chime, Chainalysis, LINE, and Intuit already trust Unit21 with their most important security processes.
To date, the company has been laser-focused on financial fraud. The product works well, and each logo it adds in the space makes it easier to acquire the next similar customer. But it’s starting to attract notice beyond that tight-knit ecosystem:
The Information recently included Unit21 in its list of 50 Most Promising Startups.
The World Economic Forum named the company a Technology Pioneer.
Twitter is working with Unit21 to power its revamped Verification process.
The company just closed a Series B from Tiger Global at a $300 million valuation.
Now, funded up and growing fast, Unit21’s job is to build out the platform, one vertical at a time, balancing flexibility and simplicity, power and ease. When it pulls it off, it will have written the playbook for building broadly-applicable tools that feel custom-built.
To understand what Unit21 is building, why it’s important, and how you might be able to wield AI/ML even if you can’t write a single line of code, we’ll cover:
Founder-Market Fit
Meet Unit21
One Platform, Many Use Cases
Initial Market: Fighting Financial Fraud
Growth and Tiger and Growth, Oh My!
When I Needed Unit21
Blue Check and Beyond
Trade-Offs and Challenges
AI/ML to the People
Unit21 exists because of a problem that both Trisha and Clarence faced: ops people aren’t empowered to write their own business logic to fight suspicious activity. The results range from wasteful to disastrous.
Founder-Market Fit
Product-Market Fit is a well-known phenomenon in Startupland. Like porn, you know it when you see it, and Superhuman CEO Rahul Vohra helped popularize a method for measuring it.
Less well-known but equally important is Founder-Market Fit, the idea that certain people are just right to start certain types of companies.
This too was defined by a certain “Je ne sais quoi” until NFX laid out four dimensions that contribute to Founder-Market Fit:
Obsession: the founders can’t stop thinking about the idea, work on it in their free time.
Founder Story: customers need to see a compelling “why” in the founders’ past.
Personality: the founders need to fit in with peers in their target market.
Experience: founders need to have just enough experience in the space, but not so much that they’re set in their ways and not open to new approaches.
Trisha and Clarence hit all four for Unit21. They have tight Founder-Market Fit.
Trisha is an experienced engineering and product leader at one of the past half-decade’s most successful startups, Affirm. Max Levchin, who founded both Affirm and PayPal, called her one of the best engineers in the country.
She first experienced the issue because Affirm has to decide whether to let any given user “Buy Now, Pay Later” in near real-time. Essentially, Affirm underwrites loans at the point of sale. Fraud is a massive risk to the company. As PYMNTS highlights, “Botching authentication methods could lead Affirm to charge either a phantom or the wrong person, having its money vanish into the ether.” So the company employs a huge team of in-house engineers and data scientists to fight fraud. They’re highly-paid talent playing table stakes defense instead of differentiated offense in a highly competitive space.
Clarence’s experience is even more personal. Like Trisha, Clarence is a genius. He grew up in Singapore and came to the US on a government scholarship awarded to the top 50 students in the country each year. He used it to study computer science and AI at Stanford, then joined Shape Security as an engineer out of school (Shape was recently acquired by F5 for $1 billion). While at Shape, Clarence got bored and started submitting papers to security conferences on the side, for fun. They were good, and conferences flew him around the world to present them. His lectures caught the attention of tech publishing powerhouse O’Reilly Media, who asked him to write a book. He did. It’s called Machine Learning and Security: Protecting Systems with Data and Algorithms.
Fun fact: Clarence is also in the GeekPwn Hall of Fame for successfully misleading a machine learning system.
One more fun fact: in 2019, Clarence became a lecturer at Berkeley in Applied Machine Learning.
OK, back to that personal experience. Shape protected large customers' websites from hackers. Part of Clarence’s job was to take descriptions of attack patterns from security operations professionals, and write rules to defend against them. One night on rotation, he took in a description, wrote a rule, deployed, and closed his computer. He got a frantic call four hours later letting him know that the client’s site had been down the entire four hours since he deployed, costing them $2 million per hour in bookings! Clarence wasn’t fired for the error, but it did light a fire in him.
He realized that the disconnect between what ops people know and what they can do is a cause of a lot of problems:
Things are lost in translation between ops and engineering.
There is a time lag between when the ops people see something and when engineers can implement a fix during which attackers can get in and wreak havoc.
Ops and engineering teams resent each other.
Even if everything worked perfectly, there just aren’t enough engineers to solve all of the challenges companies face.
Clarence left Shape in 2017. Trisha left Affirm in 2018. Both were thinking about the same problem from different angles. And both joined South Park Commons (SPC), a vibrant “community of builders, tinkerers, and domain experts” in San Francisco. SPC isn’t an incubator; it’s a place to explore new ideas before they’re anything at all. Trisha and Clarence met at SPC, and decided to explore together, specifically in the Anti-Money Laundering (AML) space.
The duo did what so many aspiring founders do: reached out to potential customers to find out if there was a “there” there. Specifically, they reached out to 300 people with “Anti-Money Laundering” in their title on LinkedIn to learn about their challenges and appetite for a solution.
One of those people was the head of AML at eBay. He told them he was doing an RFP in three weeks, and that they should submit their company. It’s just... they didn’t have a company yet.
Meet Unit21
Over those next three weeks, Trisha and Clarence did a lot:
Figured out what an “RFP” is
Incorporated Unit21, Inc.
Built a prototype
Then, they showed up to eBay’s HQ in San Jose. They brought along one of Kothari’s former Affirm colleagues to make the team look a little bigger.
The three of them presented the prototype that they’d built over the last 20 days, went home, and then heard from eBay… that they were the front-runner out of four chosen finalists. Of the other three, the smallest was a $3 billion company. Unit21 was three weeks old and worth $0 on paper.
How was three-week-old Unit21 even a contender?
In the past, there were two ways that companies built their AML/fraud models:
Built something fully custom in-house using internal engineering teams.
Bought something off the shelf from a vendor and spent half a million or so dollars to customize it.
In the latter case, companies would buy a product, integrate it into their system, tweak the inputs, and then trust a black box to spit out when a transaction was suspicious. They often erred on the side of being too cautious, sending humans a rash of false positives to sift through.
Every AML product with which Unit21 competes offers transaction monitoring that looks at a few things like who the payment is to and from, what industry they’re in, what country the payment is going to, and the volume and value of transactions. They look at the data and assign a risk score, and higher-risk transactions are sent to humans for review.
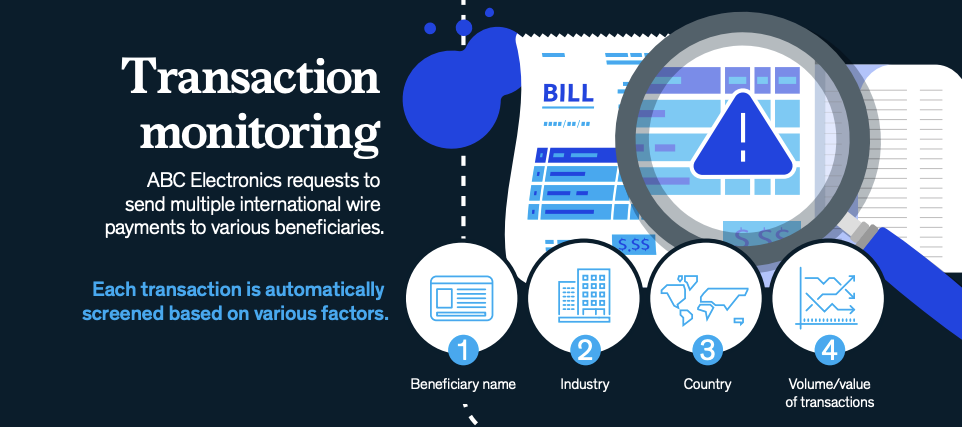
Unit21 took a new approach, one that worked well for eBay’s wide range of products, and in turn, should work for a multitude of use cases beyond financial fraud.
Instead of just monitoring the usual financial transaction data points, they told eBay, “You can give us any data and we’ll treat it like a transaction.” For example, an analyst at eBay could tag Pokémon cards by Pokémon. Unit21’s model would learn that a Charizard normally goes for $100, and flag a $1,000 Charizard purchase as anomalous. Or the system might flag a user who purchased too quickly -- if they clicked buy in one second, before having time to review anything, that might mean they didn’t actually care about the item they were buying.
What’s more, Unit21’s system wouldn’t make decisions for eBay, or spit answers out of a black box. It showed the analysts and operators the guts of the system and let them make the rules.
Ultimately, eBay told Unit21 they were too small and chose another vendor, but the process gave Trisha and Clarence confidence that they were on to something, and as they talked to more and more potential customers, they kept hearing similarly positive feedback on their approach. So they decided to go for it.
In December 2018, Unit21 raised a small seed round from Gradient Ventures and got to building. While they built, they realized that while AML was a killer use case, it wasn’t the whole product. What they were really building was a no-code tool for sophisticated business users who know how to create complex business rules or even statistical models, but can’t write or deploy code.
They were developing a new way for non-technical people to write and deploy business logic, and building out a platform, one vertical at a time.
One Platform, Many Use Cases
We’ll get to the success Unit21 has seen in its initial market in a minute, but we need to take a second to understand how they’re building what they’re building.
Clarence described it to me like this:
We want to get to the point where we’re building a single, generalized tool, but to everyone using it, Unit21 feels localized to their problem, like it was custom-built for them.
If you ask people at Unit21 what they’re creating, they might say “no-code operations tooling” or “business logic tools.” But if you ask one of the 80% of their customers who use them for AML/fraud, they would describe it as an “AML/fraud tool.” If you asked someone at Twitter, they might describe it as an “identity verification tool.”
What’s more, each AML/fraud customer’s experience with Unit21 will be different from the others, because operators and analysts write the rules and tailor it to their own needs. Ditto with identity, KYC, bookings, or any type of activity monitoring. Each team can write its own custom rules and create custom workflows, meaning that there are as many versions of Unit21 as there are Unit21 customers. Customers don’t care that there are other things that it can do; they hire Unit21 to do a specific job.
Easier said than done. How do they do it?
When Trisha and Clarence started exploring ideas together, all of their efforts went to AML/fraud. It’s what they knew. They reached out to 300 AML people. All of their conversations were about how to build a better AML/fraud product. Because they focused on building a tool, they avoided a common startup pitfall: you can’t build a platform by setting out to build a platform.
This has become a recurring theme in Not Boring: you can no longer create a successful infrastructure company by just building infrastructure directly.
Scale couldn’t just build AI infrastructure outright; they needed to start with the unsexy and manual building block: labeled data.
NexHealth couldn’t just build a new healthcare platform; they needed to sell SaaS to dentists then expand to new verticals then build APIs then build the platform.
The challenges are different for each company, and so is the approach they choose to take. Unit21’s approach is actually similar to building a marketplace.
On The Twenty Minute VC, Benchmark’s Sarah Tavel, one of the world’s best marketplace investors, talked about why chasing too big a market in the beginning is a kiss of death.
The challenge with chasing GMV [Gross Merchandise Value] is that it actually points you away from that place of dominance. Almost always, the fastest short-term path to maximizing GMV is to go after a big market, is to distract yourself by launching in multiple cities or multiple categories, and doing all those things. I kind of think of it as boiling an ocean instead of boiling a thimble. It ends up distracting you, you end up wasting resources by spreading yourself thin. So really what you have to do is stay very, very, very focused and remember the place that you're going to, which is that dominance.
It’s counterintuitive that you need to start by owning a small niche to ultimately win a massive market, but it makes sense. Startups are resource constrained. They need to hit one area that they can dominate as hard as they can. That lets them hire people who are great in that one area, build a reputation in that one area, grow via word of mouth in that one area, and solve all of the small edge case issues that add up to a great product experience in that one area. Then, and only then, do they earn the right to expand.
In Unit21’s case, the product is actually ready to handle all different kinds of data and use cases from the technical side (I’ll explain how shortly), but the company has stayed incredibly focused on its initial niche.
For Unit21, that initial niche is AML/fraud.
Initial Niche: AML/Fraud
Niches don’t have to be tiny. Financial institutions spend over $1 billion on Anti-Money Laundering tools each year, and that number is expected to grow 3-4x by the end of the decade. Last year, financial institutions around the world paid $6.8 billion in AML compliance fines.
As more commerce moves online, crypto is more heavily used, and more fintech companies steal market share from legacy financial institutions with large compliance departments, the demand for products that help companies efficiently and automatically fight financial fraud and money laundering online will continue to explode. And Unit21 is here to serve that demand.
After raising their Seed round in December 2018, Trisha and Clarence got to work turning their prototype into a working product. They launched Unit21 in July 2019 with a major first customer: Coinbase. Even Clarence admitted that they needed a “weird champion” to stick their neck out and trust a two-person team to deliver on such a critical piece of the business, and Coinbase stepped up. Then the rest came.
Here’s where focus on winning a niche kicks in: using logos to win a land-grab.
After getting a high-profile company like Coinbase on board, Unit21 was able to go to the next company, say Intuit, and say, “Look, see, Coinbase uses us for AML! You can trust us.” Then they could go to the next company, say Chime, and say, “Look, see, Coinbase and Intuit use us for AML! You can trust us.” Then they could go to the next, and the next, and the next with a growing number of logos and votes of confidence behind them.
Through the end of 2019, Unit21’s value proposition couldn’t have been more simple:
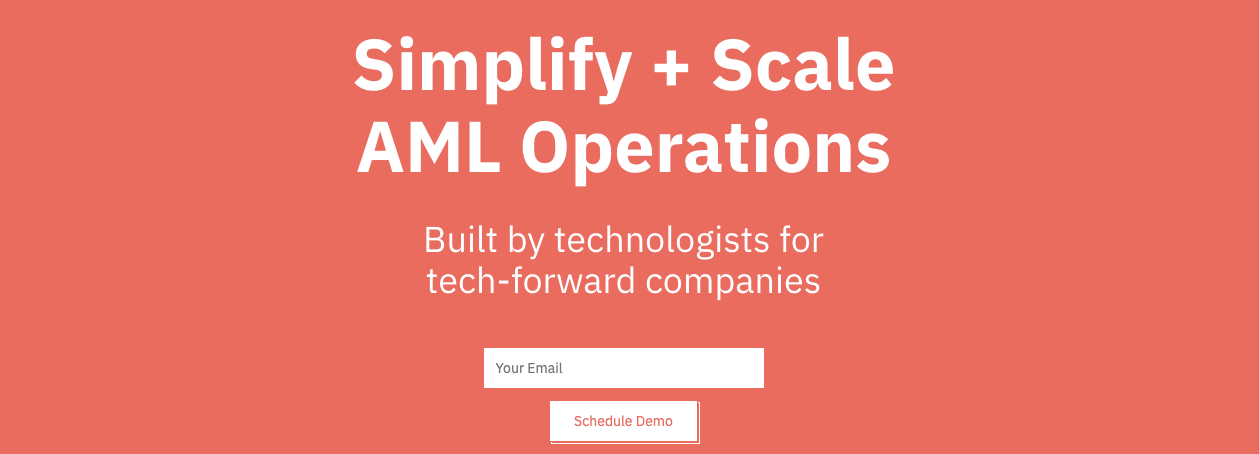
By 2020, it began slowly expanding from “AML Operations” to “Suspicious Activity,” a slightly broader category that includes AML and fraud:
Today, while the copy on the website says “The No-Code Toolset for Suspicious Activity,” Unit21 is really building infrastructure for activity monitoring. That’s a huge market, made up of a number of very big markets.
Win one niche, expand out. Win that one, expand out. It’s growth in concentric circles.
That’s how, in just two years, you go from “we can’t trust a two-person startup” to “no one ever got fired for buying Unit21” within an industry. Now, if you’re starting a neobank or fintech company, Unit21 is the default. That almost certainly wouldn’t have been the case if Trisha and Clarence had decided to boil the ocean, going after an AML customer here, a recruiting customer there, a lead gen customer there.
Today, 80% of Unit21’s customers hire the company to help with AML/fraud, and the results of that focus speak for themselves. The company’s growth was Tiger bait.
Growth and Tiger and Growth, Oh My!
One thing we haven’t really talked about yet that’s worth mentioning: Unit21 is growing absurdly fast. We focused on Founder-Market Fit, but the company does have Product-Market Fit, too. Customers are pulling Unit21.
After signing Coinbase in 2019, Clarence told me that he expected a gradual ramp-up phase, a nice clean beta period with about twenty customers, but that just never happened. Customers just kept signing up. Unit21 didn’t even have time to slow down and officially launch.
“We saw more demand than expected,” Trisha told me anti-hyperbolically. They set an ambitious goal to grow 6-7x in 2020. Then the pandemic hit, and the nine people on the team went remote. But they kept building, and the company kept growing.
In October, Unit21 raised a $13 million Series A from A.Capital Ventures. When the dust settled on 2020, the company doubled its growth goal, growing 12x. And they did it all with no marketing until December.
The growth continued early in 2021. After a strong Q1, Trisha and Clarence fielded casual conversations with VCs but weren’t actively fundraising. They hated fundraising. The previous fundraising processes resulted in good outcomes, but they were draining and distracting.
They were dreading having to run a similar process in Q2 when some noses in New York, all the way across the country, picked up the red meat-like scent of hypergrowth…
Tiger entered with a vengeance. I can give you a first-hand account of a Tiger in its natural habitat.
As Unit21 started talking to VCs after Q1, Tiger Global got in touch. Tiger had already done a lot of its due diligence upfront, and when they spoke, Tiger asked Unit21 for two things:
To send them its numbers.
A target valuation range.
The next day, Tiger sent Unit21 a term sheet to lead its $34 million Series B at a$300 million valuation, 25% above the high end of its valuation range. When Tiger wants to win a deal, it doesn’t fuck around. I asked Trisha what Tiger looked at when pricing the round, and she said it was simple:
Customer and revenue growth
Total market size
Opportunity to expand into new areas
“For everything else,” she told me, “they trust it’ll get done.”
Unit21 signed with Tiger, and ICONIQ Capital, the family office of Mark Zuckerberg, Sheryl Sandberg, Jack Dorsey, and Jeff Weiner, joined in, along with all existing investors.
Now, with a full bank account, Unit21 is ready to expand further outward from its core and attack new markets. There are more opportunities than meet the eye. I, for one, would have loved to use Unit21 when I was at Breather.
When I Needed Unit21
“If I were a prostitute, if I were dealing drugs…”
Those were the first words ever attributed to me in The New York Times.
In early October 2014, I spent hours touring our new Breather spaces with Times journalist Jonah Engel Bromwich. I was feeling good -- we’d poured blood, sweat, and tears into getting those spaces launched, and early demand and feedback were both phenomenal. Plus, it was The New York Times! My parents were going to be so proud. Bromwich and I had a long, wide-ranging conversation about real estate, the sharing economy, apps, work, and more as we trekked across the city, from building to building, together. I thought it went really well. I probably spoke over 2,000 sentences.
When the piece, This Space for Hire, hit on October 10th, I bought a physical paper, opened it excitedly, scanned quickly for my name, and found the one sentence he plucked out:
Bromwich concluded the piece pithily:
A single horror story could permanently damage the company’s reputation, thus transforming Breather into another tech-world punch line.
In the years since, I’ve learned to chalk that kind of dismissiveness up to The Times’ attitude towards tech, but Bromwich was also right. If bad things happened in our spaces, which were situated in New York City office buildings, word would spread quickly among landlords, and we wouldn’t be able to lease space. It was an existential threat.
So while some of our engineers focused on crafting the customer-facing app and web experiences, others had to spend their time creating systems and algorithms to prevent people from doing things in our spaces that were better done in the comfort of their own apartments.
First, we had to block fraudulent credit cards. As I told The Times, if you’re going to deal drugs, you don’t want anything tied back to you, so you use fake or stolen cards. Stopping bad cards was the first line of defense. That was relatively easy. We plugged in Sift Science and it did a pretty good job. Kids have it good these days; they can use Stripe Radar and stop most bad cards.
But fraudsters are creative. They’d set up accounts, then invite a bunch of dummy accounts to get free credits. They’d wait until the very last minute to make a reservation, so that we couldn’t catch them with manual review in time. They tried all manner of creative ways to beat the system.
Our plucky little team had to find ways to stop them, so we corralled engineering resources to build tools. Every week or so, me and Gio and Audrey, who ran Customer Care, got on a call with a couple of engineers to go over the new things that we’d seen on the ground, and the engineers would go back to write new ways to stop them. The best one, developed a couple years in, was called the Honey Pot. Instead of blocking suspicious users right away, we’d let them play around in the app long enough to invite their friends, and then block all of them in one fell swoop.
Most of the time, we stopped them. Sometimes, we didn’t. Often, a new trick would spread among fraudsters and they’d leverage it for a couple of weeks as we waited for our meeting with the engineers, and as they went back and coded. Occasionally, the engineers didn’t quite get what we were looking for, and would build the wrong thing. Not their fault, they weren’t the ones experiencing it and we sometimes had a hard time explaining the patterns we detected. Then, as we grew, and the existential threat hadn’t killed us yet, we got a little lax and engineers were repurposed to more important, non-ops things like trying to integrate Salesforce or Marketo for the umpteenth time.
Fraud didn’t end up killing Breather -- COVID handled that -- but we spent thousands of employee hours over many years trying to stay ahead and translate what the ops team saw into algorithms the engineers wrote, only to face new mutations, like a long, frustrating game of whack-a-mole. The ops team actually loved it, but the engineers wanted to work on things that contributed to top-line growth.
Interestingly, once we used Sift, most of the scammy behavior wasn’t on the financial side. Transaction monitoring eliminated the easy stuff, but the signals that our Ops and Customer Care teams relayed to our engineers were things like time between reservation-created-time and reservation-start-time. Plus, they changed all of the time. They were signals, in other words, that traditional AML/fraud software couldn’t help us with.
But Unit21 would have been perfect.
Amazon Recommendations for Enterprise Data
Unit21 has shown restraint in its market expansion strategy, but its product is capable of handling much more than AML, KYC, and the rest of the financial fraud acronyms. It doesn’t even know that what it’s doing is fighting financial fraud in the first place. That’s not how it’s built.
Instead of looking for very specific signs of money laundering or fraud in financial transactions, Unit21’s model takes all sorts of data, from Pokémon card prices to time between reservation made and reservation start, and finds patterns.
Clarence described what they’re building as similar to Amazon’s recommendation engine, which is now common in consumer products but hasn’t really been brought into the enterprise.
Or if you prefer, it’s on the same plane of problem that Apple faces in training models when they have no real data because customer information is private and encrypted. To make that possible, the company is working to solve some really interesting, really hard unsolved problems around training one machine learning model on different companies’ very different data.
Here’s how it works (and I’m doing my best here, this is complicated stuff!):
Unit21’s ML model starts with the understanding that all customers don’t have the same data types or store data in the same format. Even if they had the same data types, which they don’t, it would be hard to impose the same format on them. It would add friction to an otherwise smooth process. So instead, customers do their best to input schematized data, but somewhere between 50-95% comes to Unit21 in freeform.
So Unit21 trains its data models by treating them as representation models instead of supervised learning or classification models. You know what that means right? Good. Moving on.
JK. So a representation model takes in a bunch of data and figures out the relationships between everything on its own. Instead of using fields as properties, it uses them as inputs to a layer that standardizes all of it with machine learning.
Essentially, just like Amazon’s algorithms recommends flat tummy tea after you buy a jump rope because other people who bought jump ropes bought flat tummy tea, Unit21’s models can look at a bunch of messy data and pull similarities out of the jumble.
The Holy Grail here, which Unit21 is currently rolling out to a handful of customers in beta, is a a recommendation-like engine that can represent data from Chime or Chainalysis or Twitter in a standardized way.
That means that the same engine it uses to stop money launderers can be used to, say, VERIFY ME ON TWITTER @JACK!
Blue Check and Beyond
Almost a year ago to the day, I wrote a post called If I Ruled the Tweets, with some recommendations for Twitter and it’s part-time CEO, Jack Dorsey. Right at the top of the list was bringing back Verification.
I wrote:
Twitter used to verify its users - giving them a blue check on their profile - until it caused an uproar in 2017 by verifying a white supremacist. Now it only verifies some people, occasionally, through a non-public process that involves getting in touch with someone at Twitter. It’s messy.
Twitter used to run its Verification process, by which it verified that certain people were who they said they were, manually. They had a bunch of human agents reviewing every application. It was slow, expensive, and as exemplified by the 2017 white supremecist verification, error-prone. Instead of taking on the cost or headache, Twitter all but shut down the program, but that came with its own challenges. Verification is an important tool in reducing the spread of misinformation.
So recently, in May 2021 (I can neither confirm nor deny whether it was a result of my piece), Twitter announced that it was re-launching its verification process. What it didn’t announce was that the new process is powered by Unit21.
Now, instead of needing to find a connection to one of a handful of Twitter employees, anyone can apply to be verified. Twitter’s team connects a bunch of data sources to the Unit21 engine, writes and runs its own rules, and then gives a yes or no, which is reviewed by humans as a final step. If you get verified in the coming weeks, and get that shiny blue check next to your name, you’ll know who to thank.
To Twitter, Unit21 is a verification tool.
To Intuit, Unit21 is an AML tool.
To each customer, Unit21 is the activity monitoring, flagging, and review tool it needs.
Behind the scenes, it’s the exact same tool. That’s incredibly hard to pull off.
Trade-Offs and Challenges
Unit21’s success depends on pulling off a series of challenges.
On the technical side, everything I described above is challenging and cutting edge. I’m not technical enough to understand where it will fall apart, but I know that if you’re working on solving the same as-yet-unsolved problems as Amazon or Apple, success is not guaranteed.
The strategic challenge is more interesting to me, and one I think we can take lessons from. Ultimately, Unit21 is trying to eliminate a couple key trade-offs. It’s trying to:
Build a generalized product that feels localized.
Deliver an off-the-shelf product that’s highly customizable.
The former problem is a product and go-to-market challenge. Assuming that the team solves the technical challenges, it’s a matter of positioning Unit21 to an increasing number of verticals and highlighting the benefits to ops teams and analysts far beyond fintech. That’s not easy. It means that Unit21 will need to hire sales and marketing teams familiar with the experiences and pain points of ops people across verticals, and market itself in ever-broader ways while continuing to clearly express what it does.
The original tagline, “Simplify and scale AML Operations,” is an extremely clear value prop to a specific target customer.
Today’s, “The No-Code Toolset for Suspicious Activity,” requires a little more thinking on the part of a wider group of target customers.
The next iteration will necessarily be even more broad, and will need to be backed up by case studies and targeted outreach that speaks to specific localized use cases that are possible with Unit21’s generalized “flag-and-review” toolset.
The latter challenge, building an off-the-shelf product that’s highly customizable, may be an even bigger challenge.
An off-the-shelf tool might be able to handle some common needs, like flagging suspicious card activity or fake identification, but most companies will want to customize Unit21 for its own specific needs. There’s no off-the-shelf module I could have used at Breather that would have blocked certain types of accounts for making a reservation less than five minutes before start time. I would have had to build that. But I’m not technical, so even if I were able to figure out how to write the rules, I might not feel confident that I did it right. I was pretty good at Looker, but I never got into the guts and wrote anything in its language, LookML.
Unit21 will need to solve the non-trivial problem of making it easy enough for its target customers, non-technical people, to customize, while making it powerful enough to actually solve the business needs nearly as well as an in-house engineering and data science team could.
And of course, Unit21 will have to do all of that in the face of well-funded competition. While no one is taking the approach that Unit21 is, TechCrunch points out that “The space is a hot one with a number of other fraud-prevention companies raising capital in recent months, including Sift, Seon and Feedzai.” To be fair, that’s not a very nuanced take on the market. Each of those competitors takes a different approach and offers more of a point-solution. But since Unit21 is trying to win multiple markets, it will need to compete with more tailored point-solutions in each one. That’s as much an education and sales & marketing challenge as anything, but it will still present an impediment to growth.
Lastly, as Clarence pointed out to me, one of the biggest challenges Unit21 faces is how to generalize now without being overly influenced by what they’ve learned from building for the AML/fraud use case over the past year.
That said, while Unit21 plans to sell into a variety of verticals, it actually has one core target user: the operator who doesn’t like to rely on engineers to do his or her job.
AI/ML to the People
Unit21 is approaching the generalizability problem by “finding common ground truths that ops teams face today and will always face in the future, across industries.”
Chief among them, according to Clarence and confirmed by my own experience, is that operators don’t like to rely on engineers.
He felt it at Shape, where the engineers were the well-paid superstars while the ops teams were contractors who made less money. I saw it at Breather, where the Ops team had to fight for precious engineering resources and often lost out to revenue-generating teams with some new half-baked idea to grow top-line. If you’re an operator, you’ve likely felt it, too; even when you’re able to get an engineer to work on something you see as mission-critical, they’re bummed that they’re spending time working on something that’s not customer-facing.
When Unit21 is ultimately successful, this might be its greatest contribution: bringing the power of AI/ML tooling to the non-engineers who often have the most direct experience with the problem at hand. The promise of no-code/low-code isn’t just letting non-engineers design landing pages, but putting agency in the hands of operators, and filling the engineering shortfall by empowering non-engineers to solve their own problems.
As Unit21 grows, more and more of the logic of the internet will run through a tool built by a small team of Unit21 engineers and operated by front-line operators with their own needs and expertise. It’s Edge Decision Making, powered by AI.
Thanks to Prince, Trisha, and Clarence for working with me on this piece!
How did you like this week’s Not Boring? Your feedback helps me make this great.
Loved | Great | Good | Meh | Bad
Thanks for reading and see you on Monday!
Packy
Fantastic article! I am completely impressed with Unit 21's accomplishments to date and vision, too!